Building a Data Center Fit for Enterprise AI
Nov 10, 2024
TECHNOLOGY
#datacenter #enterpriseai
This article explores how businesses can build data centers optimized for the unique demands of enterprise AI. It covers key infrastructure components, design principles, and emerging technologies essential for supporting AI workloads, while addressing challenges such as scalability, security, and future-proofing.
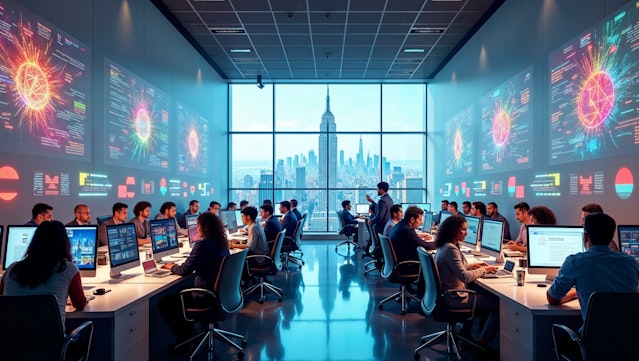
As artificial intelligence transforms the enterprise landscape, businesses are realizing that their traditional data centers may not be equipped to handle the demands of AI workloads. From training complex models to delivering real-time insights, enterprise AI places immense pressure on infrastructure. This article explores how organizations can build data centers that not only support but also enhance their AI initiatives.
Why Enterprise AI Demands a New Breed of Data Centers
AI is redefining enterprise operations, unlocking capabilities that range from predictive analytics to autonomous systems. However, these advancements come at a cost: traditional data centers struggle to meet the performance, scalability, and efficiency requirements of AI.
AI workloads require a paradigm shift in how data centers are designed and managed. Businesses must consider high-performance computing, vast amounts of data, and the agility needed to stay competitive in a rapidly evolving market.
The Foundations of an AI-Ready Data Center
Understanding AI Workloads and Their Unique Requirements
AI workloads differ significantly from traditional enterprise applications. Training AI models, for instance, demands vast computational resources, often leveraging GPUs or TPUs to handle matrix operations and deep learning tasks. Real-time AI, on the other hand, prioritizes low latency for instant decision-making.
Additionally, AI workloads are unpredictable, with data volumes growing exponentially and models evolving continuously. A data center must be built to adapt to these dynamic demands.
The Role of AI-Optimized Infrastructure
The backbone of any AI-ready data center is its infrastructure. High-performance GPUs and AI accelerators are essential for processing large-scale datasets efficiently. Storage systems need to be capable of managing high throughput while offering flexibility through tiered storage options.
Networking is another critical component. High-bandwidth, low-latency interconnects enable seamless communication between servers and devices, ensuring that AI models perform optimally, even under heavy loads.
Key Design Principles for AI-Ready Data Centers
Power and Cooling Innovations
AI infrastructure, particularly GPUs, consumes significantly more power than traditional systems. This necessitates advanced power management solutions that optimize energy use while minimizing waste.
Cooling is equally critical. Liquid cooling systems, which are more efficient than traditional air cooling, are becoming the gold standard for managing thermal loads in AI-centric data centers.
Data Management and Security
AI thrives on data, but managing massive datasets is a complex challenge. AI-ready data centers must offer robust storage solutions that facilitate fast data access while ensuring secure backups.
Security is paramount in protecting sensitive AI models and intellectual property. From encryption protocols to AI-driven threat detection systems, businesses must prioritize cybersecurity in their data center designs.
Modular and Scalable Architectures
The unpredictable growth of AI workloads demands a modular approach to data center architecture. Modular designs enable businesses to scale up or down based on need, without significant overhauls.
This approach not only saves costs but also ensures that enterprises can adapt quickly to new AI opportunities and challenges.
Integrating Emerging Technologies
Leveraging Edge Computing
As AI moves closer to end-users and devices, edge computing becomes a vital component of data center strategy. By processing data at the edge, businesses can reduce latency, improve real-time insights, and minimize the load on central data centers.
Incorporating Software-Defined Data Centers (SDDCs)
Software-defined data centers offer unparalleled flexibility. By virtualizing resources and automating management, SDDCs make it easier to allocate resources dynamically, align with AI workload demands, and optimize overall performance.
Challenges in Building AI-Optimized Data Centers
Cost and Resource Management
Building an AI-ready data center requires significant upfront investment. From procuring high-performance hardware to implementing advanced cooling solutions, costs can escalate quickly.
Businesses must balance these investments with a clear strategy for long-term ROI. Additionally, the talent gap in AI infrastructure management adds complexity, underscoring the need for specialized skills and training.
Future-Proofing AI Data Centers
AI technologies are evolving rapidly, with new hardware, algorithms, and applications emerging regularly. Future-proofing a data center involves building flexibility into its design, ensuring compatibility with upcoming innovations, and maintaining agility to pivot as needs change.
Case Studies: Successful AI-Ready Data Centers
Lessons from Industry Leaders
Enterprises like NVIDIA and Google have pioneered AI-optimized data centers, demonstrating how tailored infrastructure can drive AI success. For example, Google’s TPU pods exemplify how specialized hardware accelerates AI training at scale.
These examples underscore the importance of aligning infrastructure investments with specific business goals and AI strategies.
Conclusion
Building a data center fit for enterprise AI is no longer optional—it’s a necessity for businesses aiming to compete in the age of intelligence. By focusing on AI-optimized infrastructure, innovative design principles, and emerging technologies, enterprises can create a backbone that not only supports current AI workloads but also evolves with future demands.
The time to act is now. Enterprises that invest in AI-ready data centers today will be the ones leading tomorrow’s AI revolution.
Make AI work at work
Learn how Shieldbase AI can accelerate AI adoption.