Cheat Code to Driving AI Transformation as a Non-Technical Leader
Nov 2, 2024
ENTERPRISE
#aitransformation #leadership
Discover how non-technical leaders can successfully drive AI transformation by focusing on strategy, collaboration, and accessible tools, turning complex technology into a powerful business advantage.
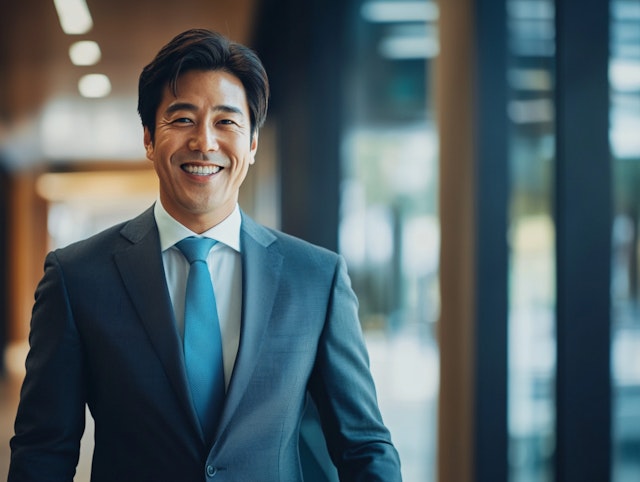
In today’s rapidly evolving business landscape, artificial intelligence (AI) is no longer optional—it's a necessity for staying competitive. However, the perception that only technical leaders can drive AI transformation often holds organizations back. The truth is, as a non-technical leader, you are uniquely positioned to steer this transformation with strategic vision and practical know-how. This article serves as your cheat code to successfully navigating AI adoption, even without a technical background.
Understanding AI Without the Jargon
What is AI, Really?
At its core, AI is about teaching machines to mimic human decision-making. This involves using data, applying algorithms, and generating outputs that can assist or automate tasks. But don’t get lost in the technicalities. Think of AI as a tool to enhance efficiency, improve customer experiences, and uncover insights.
Consider examples like predictive analytics in sales, personalized recommendations in marketing, or automated fraud detection in finance. By understanding these practical applications, you can focus on outcomes instead of the mechanics.
The Importance of Being Data-Driven
Data is the lifeblood of AI. Without high-quality data, even the most advanced AI systems will fail to deliver meaningful results. Non-technical leaders should focus on ensuring that the organization collects, cleans, and manages data effectively.
Ask questions like:
Are we capturing the right data?
How clean and consistent is our data?
Are our teams equipped to manage and analyze data effectively?
Strategic Alignment: Setting AI Goals
Tying AI Initiatives to Business Objectives
AI transformation is not about chasing the latest tech trends; it's about solving real business problems. Start by identifying areas where AI can add tangible value, such as reducing operational costs, improving customer satisfaction, or enhancing product development.
For example, if customer churn is a pain point, AI-powered predictive analytics can help you identify at-risk customers and intervene proactively. By tying AI initiatives to clear objectives, you ensure alignment with overall business goals.
Getting Buy-In from Stakeholders
AI transformation requires support across the organization. Communicate your vision in a way that resonates with stakeholders, emphasizing business outcomes rather than technical details.
For instance, instead of discussing machine learning models, highlight how AI will enable faster decision-making or boost revenue. Build cross-functional teams that include representatives from operations, IT, and other key areas to foster collaboration and accountability.
Building the Right AI Team
The Role of an AI Translator
One of the most critical roles in your AI journey is the AI translator—someone who bridges the gap between business needs and technical implementation. This person doesn’t need to be a data scientist but must understand enough about AI to guide conversations between business leaders and technical teams.
Look for professionals who excel at translating business problems into AI requirements and vice versa. They will be instrumental in ensuring your AI initiatives stay focused and relevant.
Collaborating with Data Scientists and Engineers
As a non-technical leader, you don’t need to know how to build algorithms, but you do need to create an environment where your technical teams can thrive. Encourage open communication, set clear priorities, and be prepared to iterate.
Understand that AI development is often experimental. Collaborate closely with your technical team to refine models and adjust strategies as needed.
Leveraging Tools and Frameworks
Low-Code/No-Code Platforms
Advances in low-code and no-code platforms are democratizing AI, allowing non-technical professionals to build and deploy AI solutions. Tools like these make it possible to create predictive models or automate workflows without writing a single line of code.
Explore platforms such as Google AutoML, DataRobot, or Microsoft Power Platform to see how they can fit into your organization’s AI strategy.
Partnering with AI Vendors
When working with external AI vendors, ask the right questions:
What is the expected ROI?
How do you handle data security and compliance?
What level of customization and scalability is offered?
Beware of vendor lock-in. Ensure that any solution you choose can integrate with your existing systems and adapt to future needs.
Navigating Common Pitfalls
Avoiding Shiny Object Syndrome
It’s easy to get distracted by AI trends and buzzwords, but successful transformation requires focus. Define clear KPIs for your AI initiatives and track progress regularly.
For example, if you’re deploying an AI-driven chatbot, measure success through metrics like customer satisfaction scores and average response times. Avoid implementing AI just for the sake of it; every project should serve a well-defined purpose.
Overcoming Resistance to Change
AI adoption often meets resistance from employees who fear job displacement or don’t understand the technology. Address these concerns proactively by emphasizing how AI will enhance their roles rather than replace them.
Invest in training programs to upskill employees and build an AI-friendly culture. When employees see AI as a tool for empowerment, adoption rates will increase.
Future-Proofing Your AI Strategy
Staying Ahead of AI Trends
AI is evolving rapidly, and non-technical leaders must stay informed. Read industry reports, attend conferences, and network with AI experts to understand emerging trends.
For example, keeping an eye on developments in generative AI or explainable AI can help you anticipate how these technologies might impact your industry.
Scaling AI Initiatives
As your AI initiatives gain traction, focus on scaling them across the organization. Create feedback loops to learn from early implementations and refine your approach.
Think of scaling not just in terms of technology but also people and processes. Build a governance framework to ensure AI projects align with organizational priorities and ethical standards.
Conclusion
Driving AI transformation as a non-technical leader is not about mastering algorithms—it’s about mastering strategy, collaboration, and communication. By focusing on business outcomes, building the right team, and leveraging accessible tools, you can turn AI from a complex challenge into a competitive advantage.
Start small, learn continuously, and empower your organization to embrace the AI revolution. Your leadership, not your technical expertise, is the true cheat code to success.
Make AI work at work
Learn how Shieldbase AI can accelerate AI adoption with your own data.