Data Governance: Ensuring the Integrity and Security of Institutional Information
Mar 27, 2024
ENTERPRISE
#dataprivacy #enterprise #workplace #compliance #datagovernance
In today’s data-driven world, the protection and management of institutional information are paramount for organizations across all sectors. Data governance plays a crucial role in ensuring the integrity, security, and compliance of data assets. Let’s delve into the intricacies of data governance and explore how organizations can establish robust frameworks to safeguard their data effectively.
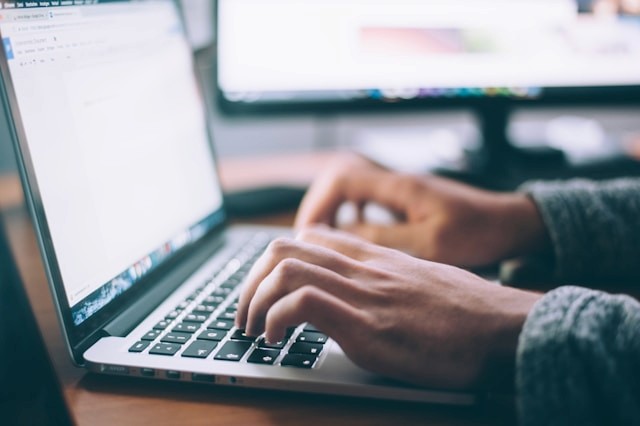
Understanding Data Governance
What is data governance?
Data governance encompasses the overall management of the availability, usability, integrity, and security of data within an organization. It involves defining policies, procedures, and roles to ensure that data is managed effectively throughout its lifecycle.
Importance of data governance in modern organizations
Data governance is essential for maintaining data quality, ensuring compliance with regulations, mitigating risks, and fostering trust in data-driven decision-making processes. It provides a structured approach to managing data assets and aligning them with organizational goals.
Key Principles of Data Governance
Data Quality Management
Data quality management is a fundamental principle of data governance that focuses on ensuring data accuracy, consistency, and reliability through standardized processes and controls. By implementing robust data quality measures, organizations can enhance the trustworthiness and usability of their data assets. Key aspects of data quality management include:
Accuracy: Ensuring that data is correct and free from errors or inconsistencies.
Consistency: Maintaining uniformity and coherence in data across different systems and sources.
Reliability: Ensuring that data is dependable and can be trusted for decision-making purposes.
Standardized Processes: Establishing clear procedures for data collection, validation, and maintenance to uphold data quality standards.
Controls: Implementing mechanisms to monitor and enforce data quality, such as data validation checks and data cleansing routines.
Data Security and Privacy
Data security and privacy are critical components of data governance that focus on protecting data from unauthorized access, breaches, and ensuring compliance with privacy regulations. Safeguarding sensitive information is essential to maintain trust with stakeholders and mitigate the risks associated with data breaches. Key elements of data security and privacy include:
Unauthorized Access: Implementing access controls, encryption, and authentication mechanisms to prevent unauthorized users from accessing sensitive data.
Breach Prevention: Employing security measures such as firewalls, intrusion detection systems, and regular security audits to prevent data breaches.
Compliance: Ensuring that data handling practices align with relevant privacy regulations (e.g., GDPR, CCPA) to protect individuals’ privacy rights and avoid legal consequences.
Privacy Regulations: Adhering to data protection laws and standards to safeguard personal information and maintain data confidentiality.
Compliance and Regulatory Requirements
Compliance with industry-specific regulations and standards is a key principle of data governance to avoid legal repercussions and maintain trust with stakeholders. Organizations must align their data management practices with regulatory requirements to uphold ethical standards and protect sensitive information. Key considerations for compliance and regulatory requirements include:
Industry Regulations: Understanding and adhering to regulations specific to the organization’s industry, such as financial regulations (e.g., SOX) or healthcare regulations (e.g., HIPAA).
Standards Adherence: Following established data governance standards and best practices to ensure data integrity and regulatory compliance.
Legal Repercussions: Recognizing the potential consequences of non-compliance, including fines, legal actions, reputational damage, and loss of stakeholder trust.
Maintaining Trust: Demonstrating a commitment to compliance and ethical data practices to build and maintain trust with customers, partners, and regulatory bodies.
Developing a Data Governance Framework
Establishing Roles and Responsibilities
Establishing roles and responsibilities is a crucial aspect of developing a data governance framework. This involves defining clear accountability for data management tasks and decision-making processes within an organization. Key points to consider include:
Accountability: Assigning specific roles to individuals or teams responsible for data governance tasks, such as data stewards, data owners, and data custodians.
Decision-Making Processes: Clarifying decision-making authority and escalation procedures for resolving data-related issues and conflicts.
Communication: Facilitating effective communication among stakeholders to ensure alignment on data governance objectives and responsibilities.
Training and Education: Providing training and resources to empower individuals in their data governance roles and responsibilities.
Creating Policies and Procedures
Creating policies and procedures is essential for establishing guidelines for data handling, access controls, and data lifecycle management within an organization. This involves documenting best practices and standards to ensure consistency and compliance. Key elements of creating policies and procedures include:
Data Handling Guidelines: Defining protocols for data collection, storage, sharing, and usage to maintain data integrity and security.
Access Controls: Establishing rules for controlling access to data based on user roles, permissions, and authentication mechanisms.
Data Lifecycle Management: Outlining procedures for managing data throughout its lifecycle, including acquisition, storage, retention, and disposal.
Documentation: Documenting policies and procedures in a clear and accessible manner to guide employees in their data governance activities.
Implementing Governance Controls and Mechanisms
Implementing governance controls and mechanisms involves leveraging technology and processes to enforce data governance policies effectively. By utilizing tools and automation, organizations can streamline governance processes and ensure compliance with established guidelines. Key considerations for implementing governance controls and mechanisms include:
Technology Solutions: Deploying data governance tools and software to automate data quality checks, monitor compliance, and track data lineage.
Process Automation: Implementing workflows and automation to streamline data governance tasks, such as data classification, metadata management, and policy enforcement.
Monitoring and Reporting: Establishing mechanisms for monitoring data governance activities, identifying issues, and generating reports to track compliance and performance.
Continuous Improvement: Iterating on governance controls and mechanisms based on feedback, emerging technologies, and evolving data governance requirements to enhance effectiveness and efficiency.
Data Classification and Sensitivity
Data classification and sensitivity are critical components of effective data governance, ensuring that organizations can protect their most valuable and sensitive information. By identifying, classifying, and safeguarding data based on its sensitivity levels, organizations can mitigate risks and maintain data integrity. Key aspects of data classification and sensitivity in data governance include:
Identifying and Classifying Sensitive Data
Identifying and classifying sensitive data is a crucial aspect of data governance that involves recognizing and categorizing data based on its sensitivity and importance. Key considerations for identifying and classifying sensitive data include:
Data Discovery: Conducting thorough assessments to identify all data assets within the organization, including structured and unstructured data.
Classification Criteria: Establishing criteria for classifying data based on sensitivity levels, such as confidential, proprietary, or regulated data.
Data Mapping: Mapping data flows and relationships to understand how sensitive data is collected, stored, and shared across systems.
Implementing Access Controls and Encryption
Implementing access controls and encryption is essential for protecting sensitive data from unauthorized access and ensuring data security. Key aspects of implementing access controls and encryption include:
Access Control Policies: Defining and enforcing access control policies to restrict data access to authorized personnel based on roles and permissions.
Encryption Protocols: Utilizing encryption algorithms to secure data both in transit and at rest, safeguarding it from unauthorized disclosure.
Data Masking: Employing data masking techniques to anonymize sensitive data for specific user roles or non-production environments.
Handling Personally Identifiable Information (PII) and Sensitive Institutional Data
Handling Personally Identifiable Information (PII) and sensitive institutional data requires organizations to adhere to strict data protection practices to safeguard individuals’ privacy and comply with regulations. Key considerations for handling PII and sensitive institutional data include:
Data Minimization: Collecting and retaining only the necessary PII required for business purposes to reduce the risk of exposure.
Data Retention Policies: Establishing data retention policies to govern the storage and deletion of PII in accordance with legal requirements.
Data Sharing Agreements: Implementing secure data transfer mechanisms and data sharing agreements when sharing PII with third parties to ensure data security and compliance.
Data Lifecycle Management
Data Acquisition and Ingestion
Data acquisition and ingestion are foundational stages in the data lifecycle management process, involving the collection and intake of data from various sources. Key considerations for data acquisition and ingestion include:
Data Collection Sources: Identifying and sourcing data from internal systems, external sources, IoT devices, or third-party sources.
Data Integration: Integrating data from disparate sources to create a unified view for analysis and decision-making.
Data Quality Assurance: Conducting data quality checks during ingestion to ensure data accuracy, completeness, and consistency.
Data Storage and Retention Policies
Data storage and retention policies are essential components of data lifecycle management, governing how data is stored, managed, and retained over time. Key elements of data storage and retention policies include:
Storage Infrastructure: Selecting appropriate storage solutions based on data requirements, such as databases, data lakes, cloud storage, or on-premises storage.
Data Backup and Recovery: Implementing backup and recovery procedures to protect data from loss, corruption, or disasters.
Retention Periods: Defining retention periods for different types of data based on regulatory requirements, business needs, and data usage patterns.
Data Archival and Disposal Procedures
Data archival and disposal procedures are critical for managing data throughout its lifecycle, ensuring that data is retained or disposed of in a secure and compliant manner. Key considerations for data archival and disposal procedures include:
Archival Strategies: Identifying data that is no longer actively used but needs to be retained for historical, compliance, or business purposes.
Archival Storage: Storing archived data in secure and accessible repositories to facilitate retrieval when needed.
Data Disposal Methods: Implementing secure data disposal methods, such as data shredding, data wiping, or data anonymization, to permanently remove data that is no longer needed.
Risk Management and Mitigation Strategies
Assessing Data-Related Risks
Assessing data-related risks is a critical component of effective risk management within data governance. Key considerations for assessing data-related risks include:
Risk Identification: Identifying potential threats and vulnerabilities that could impact data integrity, confidentiality, or availability.
Risk Analysis: Evaluating the likelihood and impact of identified risks on data assets and business operations.
Risk Prioritization: Prioritizing risks based on their severity and potential impact on the organization.
Implementing Risk Mitigation Measures
Implementing risk mitigation measures is essential to reduce the likelihood and impact of identified risks. Key aspects of implementing risk mitigation measures include:
Risk Controls: Implementing controls and safeguards to mitigate identified risks, such as access controls, encryption, and monitoring systems.
Risk Transfer: Transferring risk through insurance or contractual agreements to mitigate financial losses in the event of a data breach or incident.
Risk Avoidance: Avoiding high-risk activities or data practices that could expose the organization to significant vulnerabilities.
Incident Response and Data Breach Management
Incident response and data breach management are crucial components of risk management within data governance, ensuring that organizations can effectively respond to and recover from security incidents. Key considerations for incident response and data breach management include:
Response Planning: Developing incident response plans that outline roles, responsibilities, and procedures for responding to data breaches and security incidents.
Incident Detection: Implementing monitoring tools and systems to detect and alert on potential security incidents in real-time.
Containment and Recovery: Taking immediate action to contain security incidents, mitigate damages, and recover data and systems to normal operations.
Post-Incident Analysis: Conducting post-incident reviews to analyze the root causes of security incidents, identify areas for improvement, and enhance incident response capabilities.
Ensuring Regulatory Compliance
Relevant data protection regulations, such as the General Data Protection Regulation (GDPR) and the California Consumer Privacy Act (CCPA), play a crucial role in governing how organizations handle and protect data. Key points regarding these regulations include:
GDPR: The GDPR is a comprehensive data protection regulation that applies to organizations handling the personal data of individuals in the European Union. It sets strict requirements for data processing, consent, data subject rights, and data breach notifications.
CCPA: The CCPA is a data privacy law that grants California residents specific rights over their personal information held by businesses. It requires transparency in data collection, opt-out mechanisms, and safeguards against data breaches.
Steps for Achieving Compliance
Achieving regulatory compliance involves a series of steps to ensure that organizations meet the requirements set forth by data protection regulations. Key steps for achieving compliance include:
Data Mapping: Conducting a thorough assessment of data flows, storage locations, and processing activities to understand how data is collected, used, and shared.
Policy Development: Establishing data protection policies and procedures that align with regulatory requirements, including data retention, access controls, and data breach response.
Training and Awareness: Providing training to employees on data protection practices, privacy policies, and regulatory compliance to ensure a culture of data security within the organization.
Regular Auditing: Conducting regular audits and assessments to monitor compliance with data protection regulations, identify gaps, and implement corrective measures.
Consequences of Non-Compliance
Non-compliance with data protection regulations can have severe consequences for organizations, including financial penalties, reputational damage, and operational disruptions. Key consequences of non-compliance include:
Monetary Fines: Regulatory authorities can impose significant fines for violations of data protection regulations, such as GDPR, CCPA, or HIPAA, which can amount to millions of dollars or a percentage of annual revenue.
Operational Restrictions: Non-compliance may lead to operational restrictions, such as being barred from certain markets, facing limitations on serving customers, or being disqualified from government contracts.
Business Disruption: Non-compliance can result in work stoppages, supply chain disruptions, legal actions, and security breaches that impact daily operations and financial stability.
Reputational Damage: Violations of data protection regulations can tarnish an organization’s reputation, erode customer trust, and lead to negative media coverage, affecting long-term relationships and brand image.
Implementing Data Governance Technologies
Implementing data governance technologies involves utilizing tools and software solutions to enhance data management, compliance, and quality. Key aspects to consider include:
Data Governance Tools and Software Solutions
Data governance tools aid organizations in structuring and managing data effectively. These tools offer features like data cataloging, data lineage tracking, and metadata management to ensure data accuracy and consistency.
Automated Data Governance Workflows
Automated data governance workflows streamline processes by automating data management tasks. These workflows help in data classification, data lineage tracking, and data quality monitoring. Automation ensures efficiency, reduces manual errors, and enhances data governance practices.
Integration with Existing IT Infrastructure
Integrating data governance technologies with existing IT infrastructure is crucial for seamless operations. Compatibility with current systems, databases, and applications is essential to ensure data governance tools can effectively interact with and enhance the organization’s IT environment. Integration facilitates data sharing, data security, and compliance across different platforms.
By leveraging data governance tools, implementing automated workflows, and integrating with existing IT infrastructure, organizations can strengthen their data governance practices, ensure regulatory compliance, and optimize data management processes effectively.
Data Governance Best Practices
Data governance best practices are essential for establishing effective data management processes and ensuring data integrity. Key best practices include:
Involving Stakeholders and Fostering a Culture of Data Governance
Involving stakeholders from various departments and levels of the organization is crucial for successful data governance. Key considerations for involving stakeholders and fostering a culture of data governance include:
Executive Sponsorship: Securing executive buy-in and support for data governance initiatives to prioritize data management efforts.
Cross-Functional Collaboration: Encouraging collaboration between business units, IT teams, and data stewards to align data governance practices with organizational goals.
Training and Communication: Providing training on data governance principles and fostering open communication to promote understanding and adoption of data governance practices.
Regular Audits and Reviews
Regular audits and reviews are essential for monitoring data governance processes, identifying issues, and ensuring compliance with data policies. Key aspects of regular audits and reviews include:
Data Quality Assessments: Conducting periodic assessments to evaluate data quality, accuracy, and completeness.
Policy Compliance Checks: Reviewing adherence to data governance policies, procedures, and regulatory requirements.
Performance Metrics: Establishing key performance indicators (KPIs) to measure the effectiveness of data governance practices and track progress over time.
Continuous Improvement and Adaptation
Continuous improvement and adaptation are critical for evolving data governance practices to meet changing business needs and regulatory requirements. Key considerations for continuous improvement and adaptation include:
Feedback Mechanisms: Soliciting feedback from stakeholders to identify areas for improvement and address emerging challenges.
Benchmarking: Comparing data governance practices against industry standards and best practices to drive continuous improvement.
Agile Approach: Adopting an agile mindset to adapt data governance processes quickly in response to changing data landscapes and business requirements.
By embracing data governance principles and implementing comprehensive frameworks, organizations can safeguard their institutional information, enhance data quality, and foster a culture of trust and compliance in the age of AI.
Make AI work at work
Learn how Shieldbase AI can accelerate AI adoption with your own data.