Edge AI: Bringing AI Processing Closer to the Data Source for Real-Time Applications
Mar 19, 2025
TECHNOLOGY
#edgeai
Edge AI processes data closer to its source, enabling real-time decision-making, reducing latency, enhancing security, and optimizing bandwidth. By leveraging AI-optimized hardware, 5G, and federated learning, enterprises can unlock faster, more efficient, and privacy-conscious AI applications across industries such as manufacturing, healthcare, and autonomous systems.
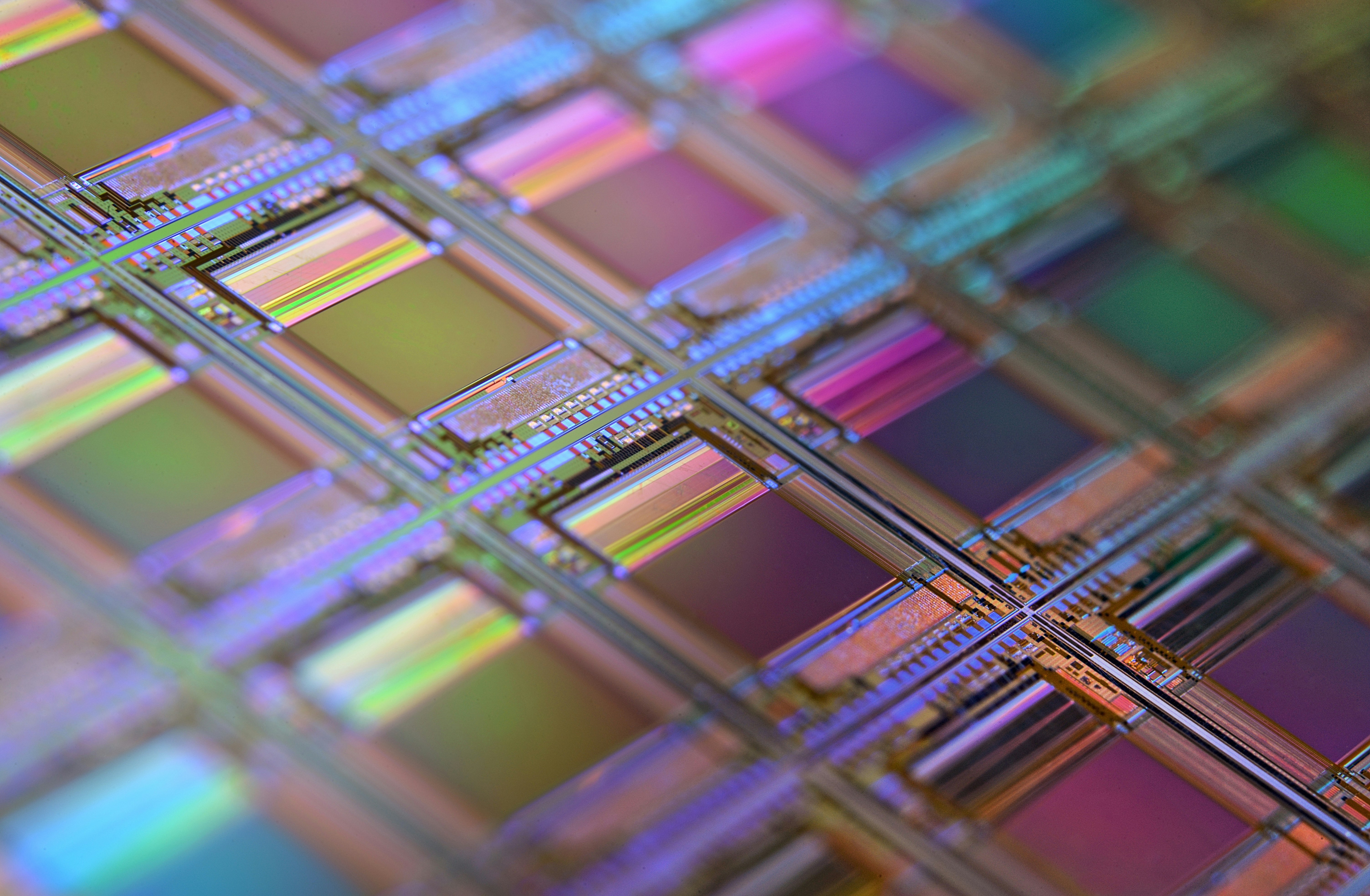
Artificial Intelligence (AI) has traditionally relied on cloud computing for processing vast amounts of data. However, as businesses increasingly require real-time decision-making and low-latency responses, cloud-dependent AI introduces challenges such as network delays, bandwidth constraints, and security risks.
Edge AI is emerging as a transformative solution, shifting AI processing closer to where data is generated—on devices, sensors, and edge servers. By processing data locally, Edge AI enables faster, more efficient, and secure AI applications, making it particularly valuable for industries where real-time insights are critical.
This article explores the concept of Edge AI, its impact on real-time applications, key enabling technologies, industry use cases, deployment challenges, and what the future holds for enterprises adopting this technology.
What is Edge AI?
Defining Edge AI
Edge AI refers to artificial intelligence models and algorithms running directly on edge devices, such as sensors, cameras, industrial machines, or smartphones, without requiring continuous cloud connectivity. This approach contrasts with traditional AI, which often depends on centralized cloud infrastructure to process data.
How Edge AI Differs from Cloud AI
Cloud-based AI requires transmitting data to remote data centers for processing, introducing latency and potential security risks. Edge AI, on the other hand, processes data at or near the source, ensuring faster response times and improved data privacy.
Key differences include:
Latency: Edge AI provides near-instant decision-making, whereas cloud AI experiences delays due to network transmission.
Bandwidth Efficiency: By reducing the need to send large datasets to the cloud, Edge AI conserves bandwidth.
Privacy & Security: Sensitive data remains on local devices, reducing exposure to cyber threats.
Offline Functionality: Edge AI can operate even in disconnected environments, ensuring uninterrupted performance.
Real-World Examples of Edge AI
Autonomous Vehicles: AI-powered cars process sensor data locally to detect obstacles and make split-second driving decisions.
Industrial IoT: AI-driven predictive maintenance on factory machines reduces downtime by identifying potential failures before they occur.
Healthcare Wearables: Smart devices analyze biometric data in real-time, alerting users and doctors about irregularities.
Why Edge AI Matters for Real-Time Applications
For industries that rely on immediate insights and automated actions, the ability to process AI workloads at the edge is a game-changer.
Reduced Latency for Instant Decision-Making
In mission-critical applications like autonomous driving, robotic automation, and emergency healthcare, even milliseconds matter. Edge AI eliminates the need to send data back and forth between the cloud and the device, enabling real-time responses.
Bandwidth Optimization and Cost Savings
Transmitting large volumes of data to cloud servers incurs high costs and can overwhelm networks. Edge AI minimizes data transmission, optimizing bandwidth usage and reducing operational expenses.
Enhanced Security and Data Privacy
Sensitive industries such as finance and healthcare must comply with stringent data privacy regulations. Processing AI workloads locally ensures that confidential data remains secure, reducing exposure to cyber threats and compliance risks.
Reliability in Disconnected Environments
Edge AI ensures continuous operations even in remote or unstable network conditions. This is particularly beneficial for industries like oil and gas, where facilities are often located in isolated areas.
Key Technologies Enabling Edge AI
Several advancements in hardware and software are making Edge AI more powerful and accessible.
AI-Optimized Edge Hardware
Companies like NVIDIA, Intel, and Qualcomm are developing AI-specific chips (GPUs, TPUs, and NPUs) designed for efficient on-device processing. These accelerators enable powerful AI workloads to run on compact, energy-efficient devices.
5G and Edge Networking
5G networks significantly improve data transmission speeds and reduce latency, making Edge AI more effective for applications that require real-time insights. The combination of 5G and Edge AI is driving innovations in smart cities, industrial automation, and telemedicine.
Federated Learning for AI Model Training
Federated learning allows AI models to be trained across multiple edge devices without centralizing data. This approach improves privacy while enabling models to continuously learn and adapt in real-world environments.
Model Compression and Optimization
AI models must be optimized to run efficiently on resource-constrained edge devices. Techniques like quantization and pruning reduce model size and computational requirements without sacrificing performance.
Industry Use Cases of Edge AI
Manufacturing and Industrial IoT
Predictive Maintenance: AI-powered sensors analyze vibrations, temperature, and other factors to predict machinery failures before they happen.
Quality Control: AI-driven visual inspection detects product defects in real-time, reducing waste and ensuring high standards.
Healthcare and Medical Diagnostics
AI-Assisted Surgery: Smart robotic systems assist surgeons with precision movements.
Wearable Health Monitoring: AI-enabled devices track vitals and detect early signs of medical conditions.
Retail and Smart Cities
Personalized Shopping Experiences: AI-powered recommendation engines analyze in-store behavior to personalize offers.
Traffic and Public Safety Monitoring: Smart surveillance systems use AI to detect anomalies and improve city security.
Autonomous Systems
Self-Driving Vehicles: Edge AI enables real-time navigation, obstacle detection, and route optimization.
Drones and Robotics: AI-driven drones execute surveillance, inspections, and deliveries without requiring constant cloud communication.
Challenges in Deploying Edge AI
Computational Constraints
Edge devices have limited processing power compared to cloud infrastructure. Running AI models efficiently on constrained hardware remains a challenge, requiring innovations in AI model optimization.
Scalability and Management
Deploying and managing AI models across thousands of edge devices in a distributed network is complex. Enterprises need robust AI lifecycle management solutions to update, monitor, and optimize models at scale.
Data Synchronization and Governance
Edge AI systems must ensure data consistency across multiple edge devices while complying with regulatory requirements such as GDPR and HIPAA. Managing decentralized data efficiently remains a critical challenge.
Security and Vulnerabilities
Edge devices, often deployed in remote or unsecured locations, are vulnerable to cyberattacks. Ensuring end-to-end security—from hardware to AI models—is essential to prevent unauthorized access and data breaches.
The Future of Edge AI
As AI adoption accelerates, Edge AI will become a cornerstone of enterprise AI strategies.
Growth Trends and Adoption Predictions
Increased investment in AI chips and edge computing infrastructure.
Expansion of Edge AI applications in critical industries such as healthcare, manufacturing, and transportation.
Convergence of Edge AI with blockchain, 6G, and quantum computing for enhanced security and performance.
Enterprise Readiness for Edge AI
To stay ahead, businesses should:
Identify AI workloads that benefit most from edge deployment.
Invest in scalable Edge AI infrastructure and lifecycle management tools.
Partner with technology providers offering AI-optimized hardware and software solutions.
Conclusion
Edge AI is revolutionizing real-time applications by bringing AI processing closer to the data source. With its ability to reduce latency, enhance security, and optimize bandwidth, Edge AI is becoming essential for industries that demand instant insights and automated decision-making.
For enterprises looking to harness the full potential of AI, integrating Edge AI into their digital transformation strategy is no longer optional—it’s a competitive necessity. By adopting the right technologies and overcoming deployment challenges, businesses can unlock new efficiencies, drive innovation, and create smarter, more responsive AI-driven ecosystems.
Make AI work at work
Learn how Shieldbase AI can accelerate AI adoption with your own data.