Fine-Tuning vs Transfer Learning
Feb 14, 2025
TECHNOLOGY
#finetuning #transferlearning #llm
Fine-tuning and transfer learning offer distinct strategies for adapting AI models to enterprise needs—fine-tuning provides high accuracy for specialized tasks, while transfer learning delivers speed and versatility for related problem domains.
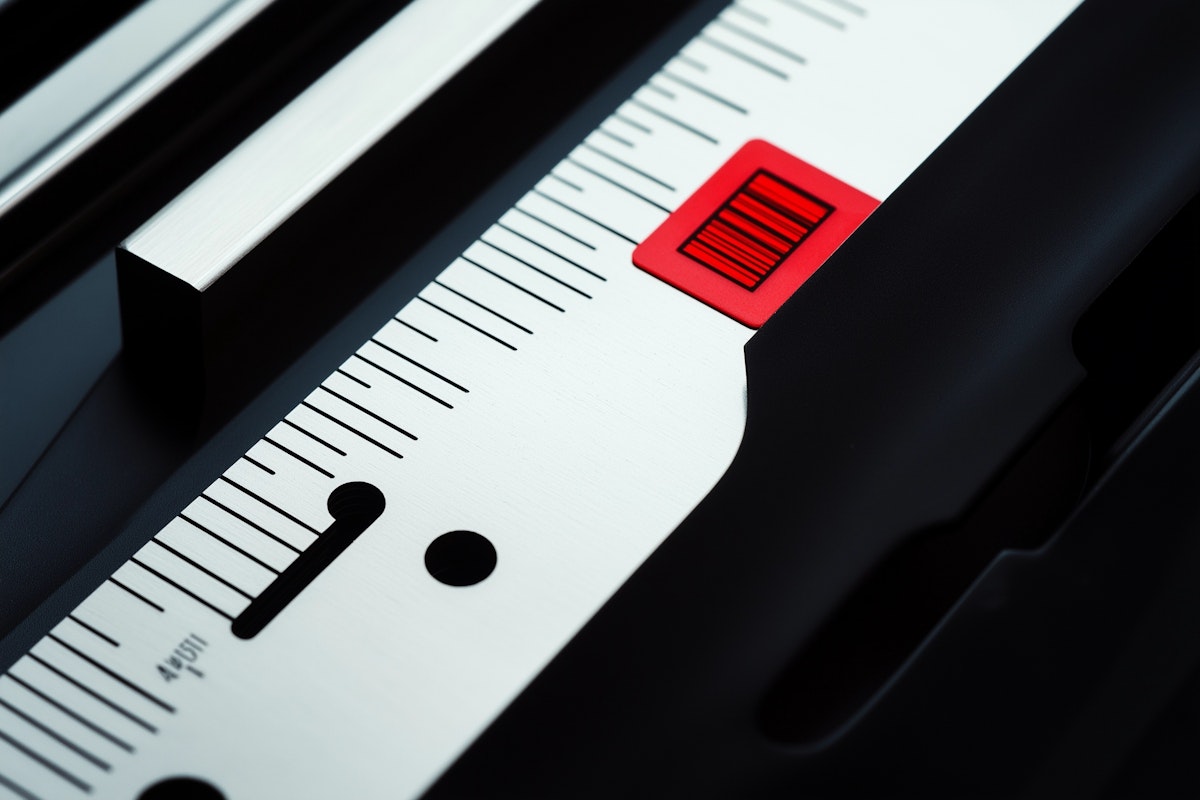
As artificial intelligence (AI) becomes a strategic asset for enterprises, choosing the right model training approach is crucial. Two powerful methods—fine-tuning and transfer learning—offer distinct advantages depending on business needs. This article explores the differences, benefits, and ideal use cases for each approach, helping business executives and professionals make informed decisions.
What is Fine-Tuning?
Definition
Fine-tuning is the process of taking a pre-trained model and adapting it to a specific task by continuing training on a new dataset. This approach modifies the model’s weights, allowing it to specialize in a particular domain.
How Fine-Tuning Works
Starts with a model pre-trained on a large dataset
Introduces a new, smaller dataset tailored to a specific task
Adjusts model parameters to refine performance
Key Benefits of Fine-Tuning
Requires less data than training from scratch
Accelerates development timelines
Offers higher accuracy for domain-specific tasks
What is Transfer Learning?
Definition
Transfer learning involves leveraging knowledge from a model trained on one task and applying it to a related but distinct task. It reuses parts of the pre-trained model, reducing the need for extensive retraining.
How Transfer Learning Works
Uses a model trained on a large, generic dataset
Applies the model to a new problem space with minimal adjustments
Maintains existing knowledge while adapting to new contexts
Key Benefits of Transfer Learning
Speeds up model training
Reduces computational costs
Provides a strong baseline with pre-trained knowledge
Fine-Tuning vs. Transfer Learning: Key Differences
Aspect Fine-Tuning Transfer Learning Purpose Specializes a model for a specific task Adapts a model to a related new task Data Requirement Needs a specific dataset Works well with limited data Flexibility High for niche applications Ideal for broader generalizations Model Adaptation Changes to model weights Reuses layers with minimal modifications
When to Use Fine-Tuning
Ideal Use Cases
When the task requires specialization, such as medical imaging or legal document analysis
When a high-quality, domain-specific dataset is available
When accuracy and attention to detail are critical
When to Use Transfer Learning
Ideal Use Cases
When adapting to a new but related problem (e.g., shifting a chatbot model from retail to healthcare)
When data is scarce
When speed to market is essential
Real-World Examples
Fine-Tuning in Action
A customer support chatbot fine-tuned with an enterprise’s historical data to provide tailored responses.
Transfer Learning in Action
A pre-trained computer vision model used for quality control in manufacturing, minimizing the need for large labeled datasets.
Challenges and Considerations
Fine-Tuning Pitfalls
Risk of overfitting if the new dataset is too small
Increased complexity in training and validation
Transfer Learning Pitfalls
Possible mismatch between the original and new tasks
Limited flexibility over the model’s architecture
Choosing the Right Approach for Your Enterprise
Key Considerations
Business Goals: Fine-tuning offers specificity, while transfer learning provides versatility.
Data Availability: Fine-tuning thrives with more data; transfer learning is ideal when data is limited.
Resources: Evaluate computational power, expertise, and time constraints.
Conclusion
Fine-tuning and transfer learning each play critical roles in enterprise AI strategies. Fine-tuning is ideal for highly specific tasks with rich datasets, while transfer learning offers efficiency and adaptability for related tasks. By aligning the choice with business goals, data availability, and resources, enterprises can effectively harness the power of AI to drive growth and innovation.
Taking the right approach now can accelerate your AI initiatives, reduce costs, and ensure your enterprise remains competitive in an increasingly AI-driven world.
Make AI work at work
Learn how Shieldbase AI can accelerate AI adoption with your own data.