How to Keep Your Data Safe When Using AI
Oct 30, 2024
TECHNOLOGY
#dataprivacy #cybersecurity
This article provides actionable strategies for businesses to protect sensitive data when integrating AI technologies. It covers essential best practices for securing data collection, storage, and AI models, while addressing AI-specific vulnerabilities, compliance with privacy laws, and ethical considerations in AI deployment.
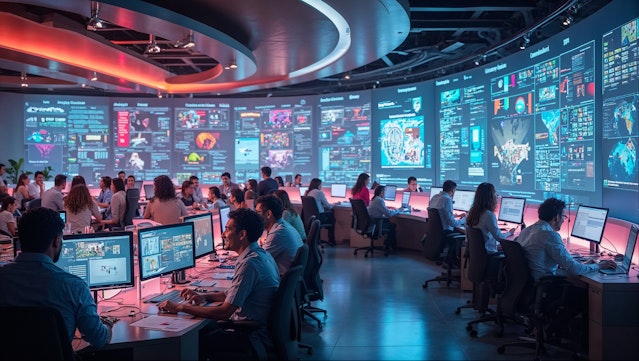
As businesses increasingly turn to AI technologies for improving efficiency, driving innovation, and gaining a competitive edge, the importance of securing sensitive data becomes more critical than ever. AI systems often handle vast amounts of data, including personal and financial information, making them prime targets for cyberattacks and privacy violations. In this article, we’ll explore how to mitigate risks to data security when using AI, providing you with practical strategies to keep your data safe.
Understanding the Risks of AI on Data Security
Data Breaches and Exfiltration
One of the most significant concerns when deploying AI systems is the risk of data breaches and exfiltration. AI models often require large datasets to function effectively, and if these models are compromised, they can lead to massive data leaks. Attackers may target AI systems directly or attempt to exploit vulnerabilities within the data pipelines, gaining access to sensitive information. Recent breaches involving AI-driven systems have highlighted the ease with which adversaries can infiltrate systems if proper safeguards are not in place.
AI-Driven Vulnerabilities
AI systems, especially those based on machine learning, are susceptible to adversarial attacks. These attacks involve manipulating the input data in ways that cause the AI to make incorrect predictions or decisions. For example, adversaries might subtly alter data inputs to mislead AI-powered security systems, causing them to fail. Moreover, AI-powered automation processes, such as those used in decision-making or business operations, can inadvertently expose vulnerabilities if the models are not thoroughly tested for robustness against such attacks.
Compliance and Privacy Concerns
The rise of AI also brings new challenges in ensuring compliance with data protection regulations such as GDPR, CCPA, and others. AI systems often process personal data at scale, and improper handling can lead to violations of privacy laws, resulting in heavy fines and reputational damage. Furthermore, AI's ability to analyze and synthesize large amounts of data can sometimes violate user privacy if not managed properly. Therefore, businesses must ensure that AI systems align with legal standards, incorporating privacy by design and data minimization practices.
Best Practices for Ensuring Data Security in AI Implementations
Secure Data Collection and Storage
The first line of defense in protecting data is ensuring that it is securely collected and stored. Sensitive data should be encrypted at rest and in transit, whether it's in a database, a cloud service, or an on-premise data center. By using strong encryption protocols, businesses can protect their data from unauthorized access, even if a breach occurs. Additionally, it's essential to establish secure data storage practices, such as regular backups, secure access controls, and audit trails, to ensure that data remains intact and accessible only to authorized personnel.
Model Security and Robustness
AI models themselves can be vulnerable to various types of attacks. It's essential to incorporate secure development practices throughout the AI lifecycle. Adversarial training, for instance, involves intentionally exposing models to attack scenarios to enhance their resilience against data manipulation. Regular testing for vulnerabilities in AI models, especially those exposed to the internet or integrated into mission-critical systems, is vital. Continuous model monitoring is also necessary to detect any irregularities that may suggest an attempt to manipulate the AI’s behavior.
Access Controls and User Authentication
Given the sensitivity of data involved in AI processes, strict access controls and robust user authentication protocols are crucial. Only authorized personnel should have access to AI systems and the data they process. Implementing multi-factor authentication (MFA) adds an extra layer of security, ensuring that even if login credentials are compromised, unauthorized access is still prevented. Furthermore, role-based access controls (RBAC) should be enforced to restrict access to sensitive data based on user roles and responsibilities.
Data Minimization and Anonymization
AI systems often require vast amounts of data, but not all of this data needs to be personal or sensitive. By implementing data minimization techniques, businesses can limit the amount of personal data they collect and use, reducing exposure to risk. Anonymization and pseudonymization are also important techniques that can help protect user identities and ensure compliance with privacy laws. Differential privacy, a technique that adds noise to data to prevent identification of individuals, is another powerful tool for safeguarding data while still enabling AI analysis.
AI-Specific Security Measures
Regular Audits and Monitoring of AI Systems
AI systems should be continuously monitored for any signs of abnormal behavior or potential security breaches. Regular audits are essential for ensuring that AI algorithms are functioning correctly and not being exploited. Automated security monitoring tools can help identify vulnerabilities in real time and trigger alerts when suspicious activity is detected. These tools also support compliance efforts by ensuring that AI systems remain aligned with data protection regulations.
AI Model Explainability and Transparency
One of the challenges of AI systems is their "black-box" nature—often, the decision-making processes within AI models are not fully transparent. This lack of explainability can pose security risks, as it becomes difficult to track how decisions are made and where vulnerabilities may lie. By incorporating explainable AI (XAI) techniques, businesses can enhance transparency, making it easier to trace how AI systems arrive at decisions. This transparency is not only essential for debugging and securing AI models but also for building trust among customers and regulators.
Incorporating Threat Detection and Response in AI
AI can also play a significant role in enhancing cybersecurity by using machine learning algorithms to detect and respond to threats. AI-driven threat detection systems can analyze patterns in network traffic, user behavior, and system logs to identify potential intrusions. By integrating AI with existing security infrastructures, businesses can improve their ability to respond to threats faster and more effectively, minimizing the impact of any potential breach.
Collaborating with Trusted Vendors and Partners
Evaluating Third-Party AI Solutions
When integrating third-party AI solutions into your operations, it's crucial to assess the security practices of vendors. Not all AI providers have the same level of commitment to data security, so it's important to ask questions about their data protection measures. Evaluate the vendor’s encryption protocols, compliance with data protection laws, and how they manage the risks associated with deploying AI systems. A thorough vetting process ensures that your data will remain secure throughout the AI implementation process.
Securing AI Supply Chains
The AI supply chain, including data sources, model development, and software components, is often complex, with multiple external parties involved. Each of these parties introduces potential security risks, whether through insecure data handling practices or vulnerable software components. To mitigate these risks, it’s essential to work with trusted suppliers and regularly audit the security of third-party solutions. Ensuring that your AI supply chain is secure helps protect your data from vulnerabilities introduced at any stage of the AI lifecycle.
Legal and Ethical Considerations
Navigating Data Protection Regulations
As AI adoption grows, so do the complexities surrounding compliance with data protection regulations. Businesses must stay up to date with evolving laws such as GDPR and CCPA, ensuring their AI systems meet the required standards. This may include implementing privacy by design, conducting data protection impact assessments (DPIAs), and establishing processes for data subject rights such as data access and deletion requests. By staying proactive in regulatory compliance, businesses can avoid costly fines and reputational damage.
Ethical Implications of AI in Data Handling
AI’s use of data raises important ethical questions about fairness, transparency, and accountability. Businesses must ensure that their AI systems do not unintentionally reinforce biases or discrimination, which can lead to negative societal impacts and legal repercussions. By embedding ethical considerations into the AI development process, including fairness audits and bias mitigation techniques, businesses can maintain the trust of their customers and comply with ethical standards.
Conclusion
The rise of AI presents enormous opportunities for businesses, but it also introduces significant risks, particularly around data security. By following the best practices outlined in this article—from securing data storage and AI models to implementing ethical data handling practices—businesses can significantly reduce the likelihood of a data breach or regulatory violation. As AI continues to evolve, so too must the strategies for securing the data that powers these systems. Prioritizing data safety will not only protect your business from security threats but will also help maintain the trust of your customers and stakeholders, paving the way for successful AI adoption and growth.
Make AI work at work
Learn how Shieldbase AI can accelerate AI adoption.