Innovation Beyond LLM Wrapper
Feb 12, 2025
INNOVATION
#llm #innovation
Discover how enterprises can move beyond traditional LLM (Large Language Model) wrappers to drive true innovation with hybrid AI models, synthetic data, and autonomous systems, unlocking new opportunities for competitive advantage and business transformation.
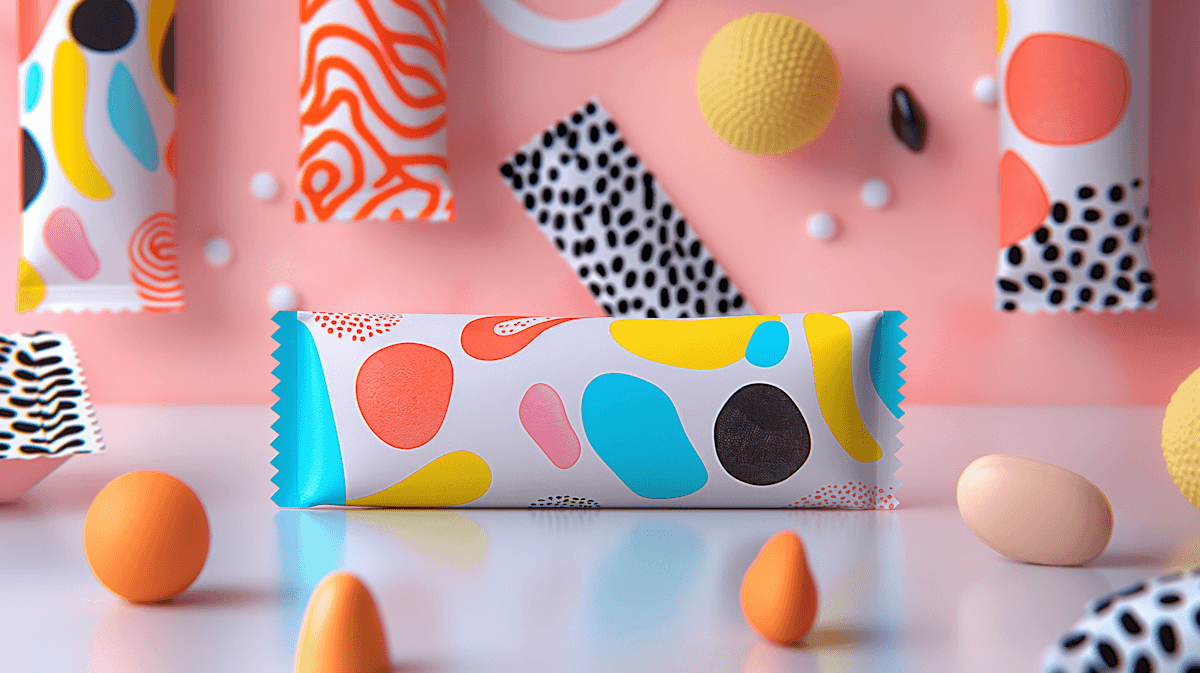
Large Language Models (LLMs) have taken the world by storm, offering powerful natural language processing capabilities that enable businesses to automate tasks, generate content, and enhance customer interactions. Traditionally, many organizations have harnessed the power of LLMs through wrappers—techniques like fine-tuning, prompt engineering, or API integrations that extend the model’s utility. While these wrappers provide quick wins and operational efficiencies, they often fall short in delivering true, long-term innovation. As the enterprise AI landscape matures, it is becoming increasingly clear that the next wave of competitive advantage lies beyond the conventional LLM wrapper.
This article explores the evolution of LLM wrappers, their limitations, and the emerging innovations that business leaders need to be aware of to stay ahead in the AI race.
The Evolution of LLM Wrappers
What Are LLM Wrappers?
LLM wrappers serve as a layer between raw language models and business applications, allowing organizations to customize and control the model’s output. They provide a practical approach to leveraging AI for specific use cases without needing to build models from scratch. Wrappers can take various forms, including:
Fine-tuning: Adjusting pre-trained models with domain-specific data
Prompt engineering: Crafting specific input prompts to guide AI responses
API integrations: Embedding LLMs into existing workflows or software platforms
Early Successes and Widespread Adoption
Initially, LLM wrappers helped businesses achieve rapid AI adoption. They offered a low-code or no-code approach to deploying advanced AI solutions, enabling industries such as customer service, marketing, and content generation to scale their operations efficiently. For example, fine-tuned LLMs helped chatbots provide more accurate responses, while prompt engineering facilitated advanced automation in copywriting and creative tasks.
However, as more enterprises adopted similar strategies, the differentiating edge of LLM wrappers began to diminish. Businesses started encountering scalability issues, inconsistencies in AI output, and challenges in maintaining quality as models evolved.
The Limitations of LLM Wrappers
Scalability and Maintenance Challenges
While LLM wrappers provide a shortcut to implementation, they often struggle with scalability. As the volume of queries or complexity of use cases increases, maintaining performance and accuracy can become difficult. Moreover, frequent updates to foundational models (e.g., new versions of GPT or BERT) can break existing wrappers, leading to maintenance overheads.
Domain-Specific Accuracy
LLM wrappers can fall short in specialized industries where domain knowledge is critical. For example, in fields like healthcare, finance, or legal, fine-tuning alone may not be sufficient to ensure reliable and compliant AI outputs. Businesses relying solely on wrappers may introduce risks related to inaccurate information, compliance breaches, or reputational damage.
Ethical and Bias Concerns
Pre-trained models, even when wrapped, can inherit biases present in their training data. LLM wrappers often lack robust mechanisms to filter out inappropriate or biased responses, which can lead to unintended consequences in customer interactions or business decisions.
Innovations in LLM Technology
Beyond Wrappers: Hybrid AI Models
To overcome these challenges, leading enterprises are exploring hybrid AI models that combine LLMs with other AI techniques, such as multi-agent systems, knowledge graphs, and symbolic AI. These approaches help mitigate the limitations of LLMs by incorporating structured data, enhancing domain-specific reasoning, and providing more controlled and transparent outputs.
Leveraging Synthetic Data
Synthetic data generation is another innovative trend helping to improve LLM performance without relying solely on traditional wrappers. By creating artificial but realistic datasets, businesses can fine-tune LLMs with higher-quality data, reducing biases and increasing accuracy in niche applications.
Embracing Multi-Modal AI
The future of LLM innovation lies in multi-modal AI, where language models are integrated with visual, audio, or sensory data inputs. This approach expands the application scope of LLMs beyond text-based scenarios, offering richer and more interactive business solutions.
Future Directions and Trends
The Rise of Autonomous AI Systems
One significant trend is the development of autonomous AI systems that do not require constant human intervention. These systems go beyond wrappers by building adaptive AI frameworks capable of learning, self-optimizing, and interacting with other AI agents to execute complex tasks.
Innovations in AI Infrastructure
To support advanced LLM capabilities, enterprises are investing in specialized AI infrastructure, including AI accelerators, edge computing, and quantum computing. These technologies not only boost processing power but also facilitate the deployment of AI models beyond traditional cloud environments.
Driving Business Strategy with Advanced LLMs
Innovative LLMs can transform business strategy by enabling predictive analytics, enhancing decision-making, and automating strategic initiatives. For executives, this translates into faster time-to-market, improved customer experiences, and more agile operational processes.
Conclusion
The age of simple LLM wrappers is coming to an end. As enterprises strive for differentiation and sustainable innovation, they must look beyond surface-level integrations and embrace advanced AI techniques. By adopting hybrid models, leveraging synthetic data, and investing in robust AI infrastructure, businesses can unlock the full potential of LLMs and drive meaningful transformation.
The future belongs to those who are not just content with applying a wrapper to existing technologies but are willing to innovate deeply, rethink AI strategies, and harness the disruptive potential of next-generation LLM solutions.
Make AI work at work
Learn how Shieldbase AI can accelerate AI adoption with your own data.