Lean Startup is Not for AI on Slow Industries
Nov 9, 2024
ENTERPRISE
#leanstartup
The Lean Startup approach, while effective in fast-paced industries, struggles to address the challenges of AI adoption in slow-moving sectors like healthcare and manufacturing. These industries require tailored strategies that prioritize compliance, reliability, and long-term pilot programs over rapid experimentation.
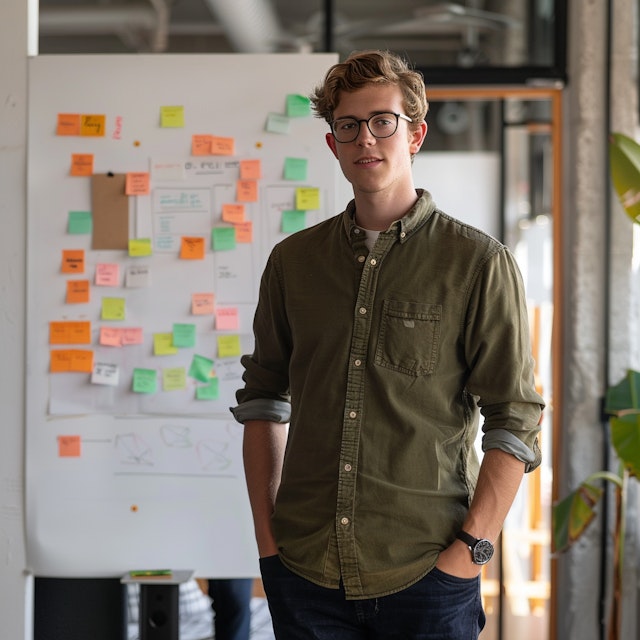
The Lean Startup methodology has revolutionized the way businesses innovate, emphasizing rapid iteration, minimum viable products (MVPs), and learning through experimentation. However, when it comes to developing and deploying AI in slow-moving industries, this framework often falls short. Industries with complex regulatory landscapes, high stakes, and slow innovation cycles demand a more nuanced approach. Let’s explore why the Lean Startup approach isn’t suitable for these sectors and what alternatives can guide AI adoption more effectively.
What Are Slow Industries?
Slow industries are sectors where innovation is inherently gradual due to factors such as heavy regulation, long approval processes, or critical safety requirements. Examples include healthcare, manufacturing, energy, and finance.
These industries often operate under strict scrutiny because errors can lead to significant consequences—ranging from financial losses to risks to human lives. The slow pace doesn’t mean these industries lack innovation; rather, they require deliberate, well-validated advancements that align with regulatory and operational standards.
Why Lean Startup Doesn’t Work for AI in Slow Industries
The Complexity of AI Solutions
AI development in slow industries is fundamentally different from traditional software development. Training robust AI systems requires extensive data collection, rigorous model training, and validation processes that can take months or even years.
Unlike a typical MVP, where initial iterations can be minimally functional, AI solutions in these sectors must perform at a near-flawless level from the outset. A faulty algorithm in healthcare could misdiagnose a patient, while an error in an industrial AI system could disrupt critical operations.
The Role of Regulation and Compliance
Slow industries are often bound by stringent regulatory requirements. For instance, healthcare AI must comply with data privacy laws like HIPAA in the United States or GDPR in Europe. Additionally, AI solutions in finance must adhere to anti-fraud and transparency guidelines.
These regulations make rapid experimentation nearly impossible. Launching an AI-driven MVP in such environments could result in non-compliance, reputational damage, or legal repercussions.
High Stakes of Failure
In slow industries, the cost of failure is often unacceptably high. A single mistake can lead to catastrophic outcomes. For example, an AI system used to monitor utility grids cannot afford to malfunction, as this could lead to large-scale outages or safety hazards.
This high-risk environment is incompatible with the trial-and-error ethos of the Lean Startup methodology. Stakeholders demand certainty and reliability, which requires a more methodical approach to product development.
Alternative Frameworks for AI in Slow Industries
Agile + Stage-Gated Approaches
A hybrid approach combining agile development with stage-gated processes can be a more effective model. Agile allows for iterative development, while stage gates provide structured checkpoints to ensure each phase meets regulatory and operational criteria.
For instance, an AI project in energy management might go through distinct stages: data validation, initial model testing, regulatory approval, and finally, phased deployment.
Pilot Programs Over MVPs
Instead of launching MVPs, businesses in slow industries should focus on long-term pilot programs. These pilots allow companies to test AI systems in controlled environments, ensuring performance and compliance before broader rollout.
An example is the use of AI for predictive maintenance in manufacturing. Rather than deploying a half-finished model across all operations, companies can test it on a single production line, collect results, and refine the system before scaling.
AI Readiness Assessments
Before diving into AI development, organizations should assess their readiness. This includes evaluating data quality, infrastructure, and workforce capabilities. AI readiness assessments help identify gaps and ensure that projects are built on a strong foundation.
A readiness-focused approach prevents rushing into development without understanding the complexities involved, reducing the likelihood of costly missteps.
Balancing Innovation and Caution
AI adoption in slow industries requires a careful balance between innovation and caution. Stakeholder involvement is critical from the beginning. Regulatory bodies, industry experts, and end-users should be engaged early to align on goals and requirements.
Organizations must also foster a culture of innovation that doesn’t compromise safety or quality. This can be achieved by building cross-functional teams that include compliance officers, domain experts, and AI specialists.
Key Takeaways for AI Leaders
Understand the limitations of the Lean Startup methodology in regulated, high-stakes environments.
Adopt tailored frameworks, such as combining agile development with structured stage gates, to suit industry-specific challenges.
Prioritize pilots and readiness assessments to mitigate risks and ensure compliance.
Foster collaboration across technical and regulatory teams to achieve both innovation and reliability.
Conclusion
While the Lean Startup approach has proven invaluable in fast-moving industries, it is ill-suited for AI projects in slow industries. The unique challenges of these sectors require a more deliberate, structured approach that emphasizes reliability, compliance, and stakeholder trust. By adapting strategies to meet these needs, businesses can unlock the transformative potential of AI while navigating the complexities of their operating environments.
Make AI work at work
Learn how Shieldbase AI can accelerate AI adoption.