Making the Case For Fine-Tuning
Jan 12, 2025
TECHNOLOGY
#finetuning
Fine-tuning can be a strategy for enterprises to maximize the value of AI. By adapting pre-trained models to specific business needs, organizations can achieve cost-effectiveness, scalability, and a competitive edge while addressing challenges like data requirements and integration complexities.
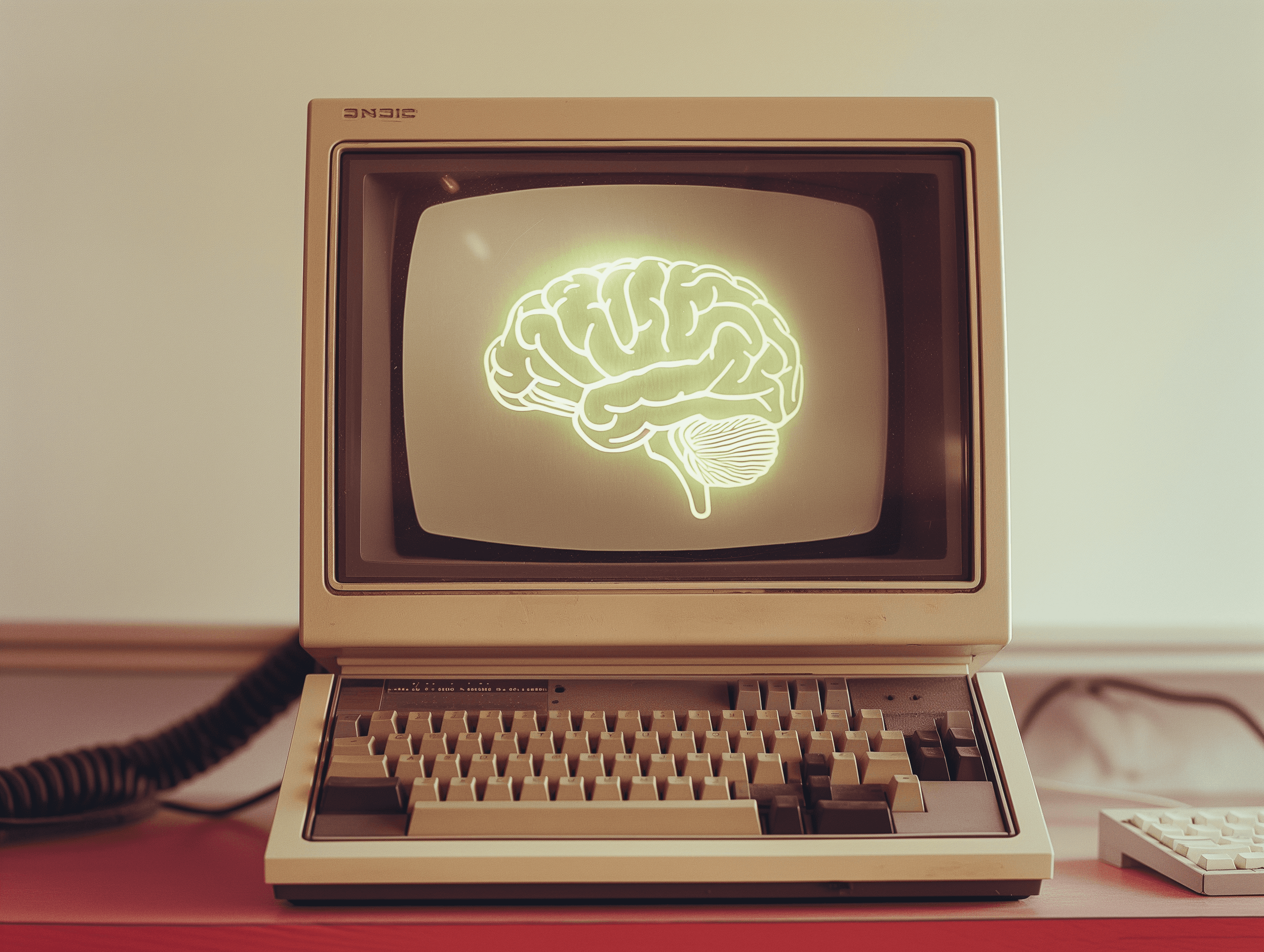
Artificial Intelligence (AI) is transforming how enterprises operate, driving efficiency, and unlocking new opportunities. However, pre-trained models often fall short when faced with specific business needs. Fine-tuning—the process of adapting a general-purpose AI model to address domain-specific challenges—offers a solution that bridges this gap effectively. This article explores why fine-tuning is a critical strategy for businesses aiming to maximize the value of AI.
Understanding Fine-Tuning
Fine-tuning is the process of taking a pre-trained AI model and refining it using domain-specific data. This involves adjusting the model’s parameters to improve its performance in targeted applications. Unlike building models from scratch, fine-tuning leverages the foundational knowledge already embedded in pre-trained models, enabling faster and more cost-effective customization.
For enterprises, fine-tuning is essential because generic models often fail to meet the nuanced demands of specialized industries. A healthcare company, for example, might need a model to interpret medical data, while a financial institution might require a system for detecting complex fraud patterns. Fine-tuning ensures these needs are addressed with precision.
The Business Case for Fine-Tuning
Fine-tuning is more than just a technical enhancement; it’s a strategic advantage. Here are the key reasons why businesses should invest in this approach:
Cost-Effectiveness
Building an AI model from scratch can be prohibitively expensive and time-consuming. Fine-tuning significantly reduces these costs by starting with a pre-trained foundation. This accelerates deployment and minimizes resource requirements.Scalability
Fine-tuned models can be tailored for specific departments, teams, or use cases, making it easier to scale AI solutions across an organization. For example, a company could fine-tune separate models for customer service, supply chain optimization, and marketing.Competitive Edge
Fine-tuned models deliver outputs that are more relevant and accurate, enabling businesses to offer differentiated services. This capability can set a company apart in competitive markets.Regulatory Compliance and Ethical Alignment
Many industries face strict regulatory requirements. Fine-tuning allows organizations to incorporate compliance measures directly into their models. Additionally, it ensures AI systems align with corporate values, reducing the risk of ethical missteps.
Key Use Cases for Fine-Tuning in Enterprise AI
Fine-tuning has transformative potential across various industries. Here are some notable applications:
Customer Support
Enterprises can fine-tune AI chatbots to handle industry-specific terminology and address customer queries with greater accuracy.Finance
Financial institutions can fine-tune models to detect anomalies and prevent fraud by analyzing their unique transactional data.Healthcare
By fine-tuning models with medical datasets, healthcare providers can enhance diagnostics, treatment recommendations, and patient care.E-commerce
Personalized recommendation engines can be fine-tuned to match consumer preferences, improving customer engagement and boosting sales.
Addressing Challenges
Despite its advantages, fine-tuning comes with challenges that businesses must navigate:
Data Requirements
High-quality, domain-specific data is essential for effective fine-tuning. Organizations need robust strategies for collecting, cleaning, and managing such data.Costs and Resources
While less expensive than building from scratch, fine-tuning still requires investment in expertise, computing power, and ongoing maintenance.Integration Concerns
Fine-tuned models must align with existing systems and workflows, which can be complex in large enterprises.
Implementation Strategy
To implement fine-tuning effectively, businesses should consider the following strategies:
Adopt Best Practices
Collaborate with domain experts to ensure models capture the nuances of specific industries. Regularly evaluate and retrain models to maintain performance.Choose the Right Technology Stack
Determine whether to use cloud-based platforms or on-premises infrastructure for fine-tuning workflows, depending on organizational needs.Explore Collaboration Models
Partnering with AI service providers or building in-house capabilities can both be viable approaches, depending on resource availability and strategic goals.
The Future of Fine-Tuning in Enterprise AI
The role of fine-tuning in enterprise AI is poised to grow. Emerging trends include the rise of low-code/no-code platforms that simplify the fine-tuning process and the integration of fine-tuned models with edge computing for real-time decision-making. Over the long term, fine-tuning will likely become a standard practice, making AI customization accessible to a broader range of organizations.
Conclusion
Fine-tuning represents a powerful opportunity for enterprises to unlock the full potential of AI. By addressing domain-specific challenges, improving scalability, and ensuring compliance, it positions organizations to thrive in competitive and dynamic markets. Investing in fine-tuning today is an investment in long-term success, enabling businesses to stay ahead in the AI-driven future.
Make AI work at work
Learn how Shieldbase AI can accelerate AI adoption.