RAG vs RIG
Oct 23, 2024
TECHNOLOGY
#rag #rig
RAG (Retrieval-Augmented Generation) and RIG (Retrieval-Integrated Generation) are two AI paradigms that enhance large language models by incorporating external or domain-specific data. RAG excels in dynamic, modular setups for real-time adaptability, while RIG offers tightly integrated systems for superior contextual understanding, ideal for specialized or static domains.
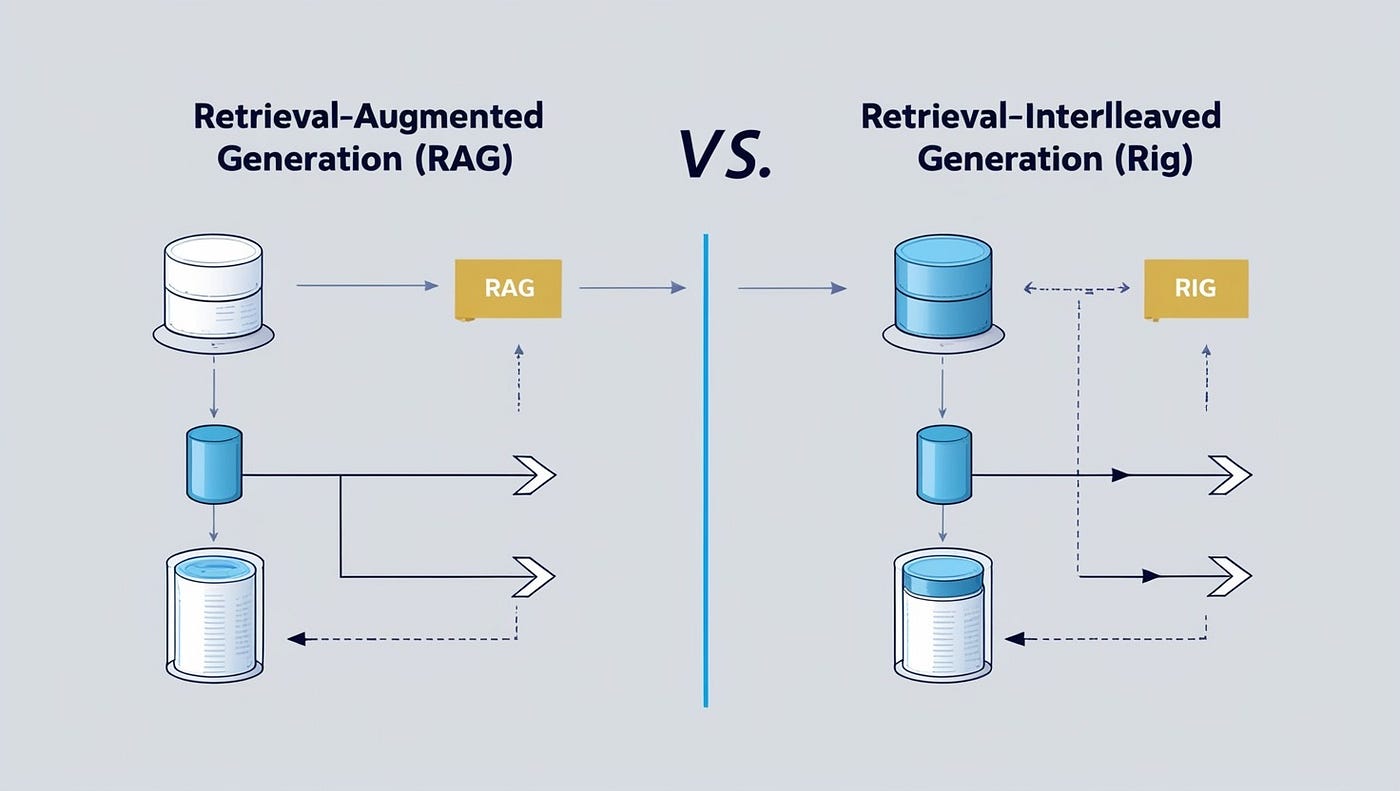
In the evolving landscape of enterprise AI, businesses constantly seek solutions that balance precision, scalability, and relevance. Two dominant paradigms have emerged to address these needs: Retrieval-Augmented Generation (RAG) and Retrieval-Integrated Generation (RIG). Both approaches aim to enhance the capabilities of large language models (LLMs) by integrating external or domain-specific data, but they do so in fundamentally different ways.
This article delves into the nuances of RAG and RIG, providing a comparative analysis to help executives and professionals make informed decisions about which approach best aligns with their enterprise needs.
Understanding RAG: A High-Level Overview
What is RAG?
Retrieval-Augmented Generation is an AI paradigm that combines LLMs with external knowledge retrieval systems. In this setup, the LLM retrieves relevant data from external sources—such as a database or document repository—and uses it to generate responses.
Key Components of RAG:
Knowledge retrieval: A mechanism to fetch relevant documents or data points.
Integration with an LLM: The retrieved data is passed to the language model to produce an informed response.
RAG Use Cases:
Customer Support Automation: Enhances chatbots by integrating dynamic FAQ retrieval.
Legal Document Review: Assists legal teams by fetching relevant precedents or clauses.
Real-Time Market Analysis: Provides contextually rich insights based on the latest financial data.
RAG's modularity makes it a versatile tool for enterprises that rely on ever-changing datasets and require real-time adaptability.
Understanding RIG: A High-Level Overview
What is RIG?
Retrieval-Integrated Generation takes a more unified approach. Here, the retrieval and generation mechanisms are tightly coupled within the same AI model. This approach often involves pre-training the model with both domain-specific and general-purpose data, resulting in a cohesive system that excels in maintaining context.
Key Components of RIG:
Unified architecture: Retrieval and generation operate within the same framework.
Domain-specific training: The model is fine-tuned on relevant data for enhanced contextual accuracy.
RIG Use Cases:
E-commerce Personalization: Offers tailored product recommendations by deeply understanding user preferences.
Healthcare Decision Support: Delivers critical insights while maintaining strict contextual fidelity.
Real-Time Context-Aware Systems: Powers applications requiring high coherence, such as financial modeling.
While RIG offers superior contextual understanding, it requires a significant upfront investment in model training and fine-tuning.
Comparing RAG and RIG
1. Architecture and Design:
RAG: A modular setup where retrieval and generation are separate, making it easier to customize.
RIG: A monolithic architecture that deeply integrates retrieval and generation.
2. Performance and Accuracy:
RAG: Can struggle to maintain continuity across multiple retrievals.
RIG: Provides enhanced contextual accuracy, especially for complex, multi-turn interactions.
3. Scalability and Maintenance:
RAG: Easier to update due to its decoupled design; individual components can be modified independently.
RIG: Requires re-training or significant adjustments to the entire system when updates are needed.
4. Latency:
RAG: Retrieval from external sources can introduce delays.
RIG: Tends to have lower latency since the model performs all processing internally.
5. Cost Considerations:
RAG: Generally lower upfront costs but can incur higher operational expenses due to ongoing retrieval needs.
RIG: Demands a higher initial investment but offers long-term cost efficiencies for static or semi-static domains.
Choosing the Right Approach
Key Decision Factors:
Use Case: RAG is better for dynamic, data-intensive scenarios, while RIG excels in static or highly specialized domains.
Budget: Enterprises with limited budgets may prefer RAG for its modularity, whereas those with larger budgets can benefit from RIG's contextual depth.
Data Specificity: RIG is ideal for industries with strict compliance requirements, such as healthcare or finance.
Latency Tolerance: For applications needing real-time performance, RIG may be the superior choice.
Enterprise Examples:
RAG: A multinational consulting firm uses RAG to equip its consultants with on-the-fly access to proprietary knowledge bases.
RIG: A healthcare organization employs RIG for advanced diagnostics, ensuring every insight is deeply contextualized.
Future Trends in Retrieval-Based AI Systems
Hybrid Models:
Emerging systems are beginning to combine the modularity of RAG with the contextual depth of RIG, offering the best of both worlds.
Real-Time Data Streams:
As enterprises increasingly rely on real-time analytics, the demand for systems that integrate live data into their responses will grow, potentially favoring RAG in dynamic environments.
Enterprise AI Ecosystem Shifts:
As AI ecosystems mature, we may see the adoption of RIG grow in regulated industries, while RAG continues to dominate in fast-moving, data-heavy fields like media and marketing.
Conclusion
RAG and RIG represent two distinct approaches to solving the challenges of enterprise AI, each with its strengths and trade-offs. RAG offers flexibility and adaptability, making it ideal for dynamic, real-time use cases. RIG, on the other hand, provides unparalleled contextual understanding and coherence, suitable for industries with specific, high-stakes needs.
For business leaders, the decision should align with the enterprise's strategic priorities, available resources, and the nature of its AI use cases. Whether your organization chooses RAG or RIG, the ultimate goal is to unlock the full potential of AI to drive innovation and efficiency.
Make AI work at work
Learn how Shieldbase AI can accelerate AI adoption.