Reinforcement Learning vs Imitation Learning
Feb 17, 2025
TECHNOLOGY
#llm
Reinforcement Learning (RL) and Imitation Learning (IL) offer distinct approaches for enterprise AI, with RL excelling in dynamic environments through trial and error, while IL efficiently replicates expert behavior in safety-critical and regulated industries; understanding their differences helps business leaders choose the right strategy for their AI initiatives.
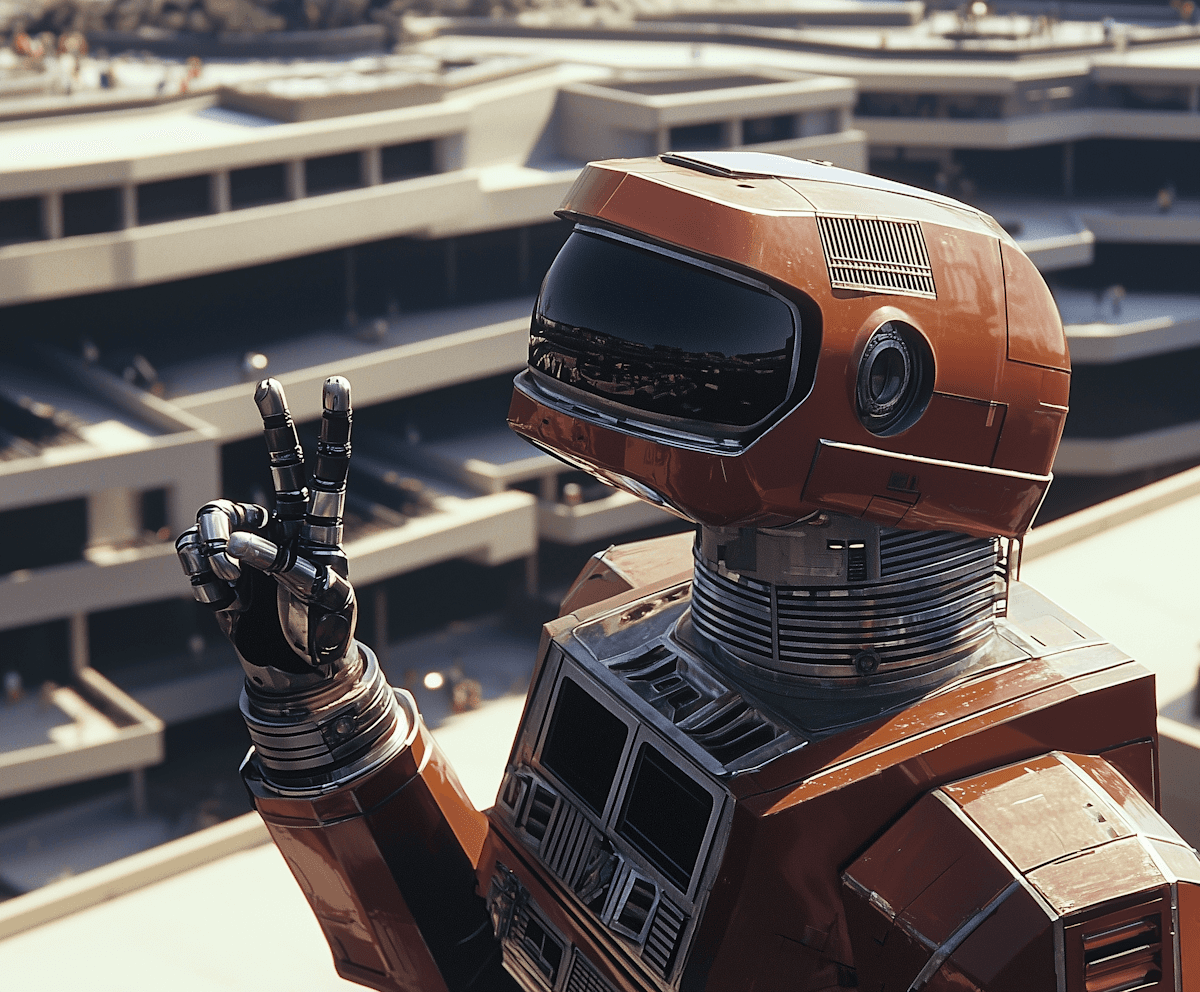
As enterprises continue their digital and AI transformation journeys, selecting the right machine learning approach can significantly impact business outcomes. While supervised and unsupervised learning are well-known paradigms, advanced AI initiatives often require more sophisticated techniques, such as Reinforcement Learning (RL) and Imitation Learning (IL). Both approaches offer distinct advantages and are suitable for different scenarios, but choosing the wrong one can lead to costly inefficiencies.
This article explores the differences between RL and IL, their respective use cases, and offers guidance to business executives and professionals on when to adopt each approach to accelerate enterprise AI success.
What is Reinforcement Learning?
Definition and Core Principles
Reinforcement Learning is a type of machine learning where an agent learns by interacting with its environment, making decisions, and receiving feedback through rewards or penalties. The objective is to learn a strategy (policy) that maximizes cumulative rewards over time.
How Reinforcement Learning Works
Agent: The AI system that makes decisions.
Environment: The domain in which the agent operates.
Action: Choices the agent can make.
Reward: Feedback on the quality of the agent’s actions.
By continuously exploring and exploiting different strategies, the RL agent refines its decision-making process, becoming more effective in complex environments.
Enterprise Use Cases for Reinforcement Learning
Autonomous Decision-Making: Robotics and automated manufacturing.
Dynamic Pricing: E-commerce and hospitality industries.
Resource Allocation: Supply chain optimization and energy management.
What is Imitation Learning?
Definition and Core Principles
Imitation Learning involves training an AI model to mimic the behavior of human experts by learning from demonstrations. Unlike RL, which relies on trial and error, IL leverages historical data and expert guidance to achieve desired outcomes.
How Imitation Learning Works
Demonstrations: Expert-provided examples of how tasks should be performed.
Behavior Cloning: Replicating expert actions directly.
Inverse Reinforcement Learning: Deriving objectives by observing expert behavior.
Enterprise Use Cases for Imitation Learning
Process Automation: Automating repetitive tasks such as data entry and customer support.
Safety-Critical Applications: Healthcare diagnostics and financial analysis where expert oversight is vital.
Training AI Systems: Autonomous driving, where models learn from experienced human drivers.
Key Differences Between Reinforcement Learning and Imitation Learning
Criteria Reinforcement Learning Imitation Learning Learning Approach Trial and error with rewards Mimicking expert behavior Data Requirements Requires interaction with environments Needs high-quality demonstrations Training Complexity Potentially long training cycles Faster to implement Real-World Applicability Suitable for dynamic environments Ideal for replicating known tasks
When to Use Reinforcement Learning in Enterprises
High Complexity and Dynamic Environments
RL is particularly useful in scenarios where the environment changes rapidly, and the AI needs to adapt through continuous learning.
Exploring New Strategies
When enterprises are pioneering new business models or optimizing dynamic systems, RL can help discover innovative strategies through experimentation.
Example Use Cases
Logistics Optimization: Adapting delivery routes in real-time.
Financial Trading: Building AI-driven trading strategies in volatile markets.
When to Use Imitation Learning in Enterprises
Leveraging Expert Knowledge
If an enterprise has access to high-quality expert demonstrations, IL can quickly replicate successful behaviors, reducing the need for extensive data collection and training.
Ensuring Safety and Compliance
IL is a strong choice in regulated industries where safe and predictable AI behavior is crucial.
Example Use Cases
Customer Service Automation: Mimicking the responses of top-performing agents.
Medical Diagnostics: Learning from the diagnostic decisions of seasoned healthcare professionals.
Challenges and Considerations
Reinforcement Learning
High Data and Computational Needs: RL requires significant computational power and time to train effectively.
Risk of Suboptimal Behavior: During the learning phase, the AI might make poor decisions that could negatively affect operations.
Imitation Learning
Dependent on Expert Demonstrations: IL is only as good as the quality of the data provided.
Limited Adaptability: Unlike RL, IL may struggle to handle scenarios not covered by the initial demonstrations.
Choosing the Right Approach for Your Enterprise AI Strategy
Key Factors to Consider
Data Availability: RL needs vast amounts of interaction data, while IL needs expert demonstrations.
Training Time and Costs: IL often has a quicker turnaround than RL.
Environmental Complexity: RL is better for dynamic systems; IL is ideal for structured, repeatable tasks.
Compliance and Safety: IL can provide a safer approach in highly regulated industries.
Decision-Making Framework
When selecting between RL and IL, executives should evaluate their specific use case against the criteria mentioned above. Developing a simple decision matrix or consulting with AI experts can help determine the optimal path.
Conclusion
Reinforcement Learning and Imitation Learning each offer unique benefits to enterprises seeking to leverage AI for business growth. By understanding the key differences and evaluating the specific needs of your business, you can choose the right approach that aligns with your enterprise AI strategy. Whether optimizing logistics, enhancing customer service, or innovating through automation, selecting the appropriate learning method can provide a strategic advantage in today's competitive landscape.
Executives and professionals should assess their data, resource availability, and industry requirements to make an informed decision that drives enterprise AI success.
Make AI work at work
Learn how Shieldbase AI can accelerate AI adoption.