Selecting Winning Stocks with AI
Dec 30, 2024
INNOVATION
#stock #capitalmarket
Artificial intelligence (AI) is revolutionizing the way we select stocks. By automating data analysis and pattern recognition, AI empowers investors to make more informed and profitable decisions. From machine learning to natural language processing, AI algorithms analyze vast datasets, predict market trends, and uncover hidden opportunities. This guide explores the potential of AI in stock selection, its applications, and the future of this exciting field.
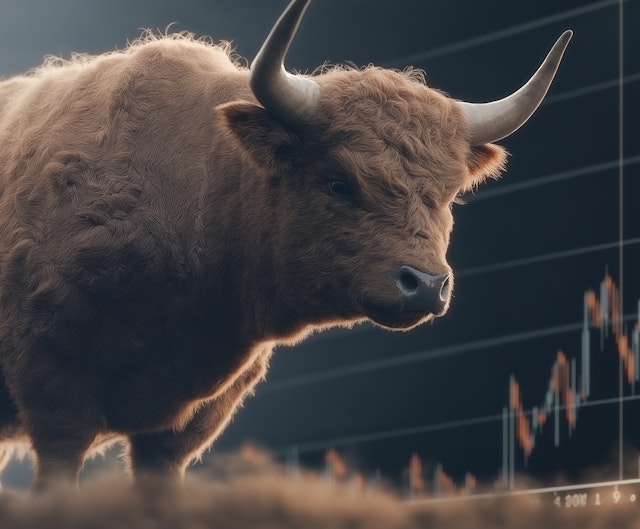
The process of selecting winning stocks has long been a critical aspect of financial success, traditionally relying on a mix of human intuition, market research, and technical analysis. However, the advent of artificial intelligence (AI) has introduced a powerful new dimension to this field, offering businesses and professionals a sophisticated toolset for making more informed investment decisions. In this article, we’ll explore how AI can be leveraged to enhance stock selection, the types of AI models involved, the data requirements, and the real-world applications that are transforming finance today.
Understanding AI in Stock Selection
How AI Enhances Traditional Stock Picking Methods
Historically, stock selection has been a labor-intensive and subjective process. Financial analysts would sift through vast amounts of data to find trends and insights. Artificial intelligence changes this paradigm by automating the analysis process, significantly improving speed and accuracy. AI algorithms excel at processing large datasets, recognizing patterns, and predicting future outcomes. They can analyze millions of data points in seconds, capturing subtle, non-obvious connections that human analysts might miss.
Types of AI Models Used in Stock Selection
AI-driven stock selection primarily involves three types of models:
Machine Learning (ML) Models: These models learn from data to make predictions. Supervised learning, for example, uses historical stock prices and company fundamentals to predict future movements. Unsupervised learning is used to identify patterns in market data that are not labeled, which can uncover hidden opportunities. Reinforcement learning helps AI agents learn from their actions over time, making decisions that improve over time.
Natural Language Processing (NLP): NLP is crucial for analyzing financial news, earnings calls, and social media sentiment. By understanding textual data, AI can gauge market sentiment and the potential impact on stock prices. This capability allows investors to incorporate real-time news into their trading decisions, potentially gaining an edge in fast-moving markets.
Deep Learning: This involves neural networks to model complex, non-linear relationships in financial data, such as identifying patterns in stock charts and technical indicators. Deep learning models can process vast amounts of historical price data to recognize trends and correlations, making them invaluable for technical analysis.
Data Requirements for AI-Driven Stock Selection
Quality and Sources of Data
For AI to be effective in stock selection, the quality of data is paramount. High-quality, clean, and reliable financial data is essential. Data sources typically include financial statements, stock price histories, economic indicators, and market news. The integration of alternative data sources, like satellite imagery for commodity predictions or foot traffic for retail stocks, is also becoming more common. These data sources provide a more comprehensive view of market dynamics, enabling AI models to make more accurate predictions.
Data Preprocessing
Before AI models can be trained, the data must undergo preprocessing. This involves cleaning and normalizing data to remove inconsistencies and irrelevant information. Feature engineering plays a critical role here, transforming raw data into meaningful features that the AI models can use. Techniques like data normalization, outlier detection, and dimensionality reduction are crucial to improving model performance.
AI Techniques for Stock Selection
Backtesting AI Models
One of the key steps in integrating AI into stock selection is backtesting. Backtesting involves applying the AI model to historical data to see how well it would have performed if those historical conditions were real-time. This process helps identify the strengths and weaknesses of an AI-driven strategy and refine it accordingly. However, it also comes with challenges, such as avoiding data leakage — where information from future events contaminates the training process.
AI Model Evaluation
Evaluating AI models is critical for ensuring their effectiveness. Metrics like accuracy, precision, recall, F1 score, and the Sharpe ratio are commonly used to assess model performance. These metrics help determine the quality of predictions and the risk-return profile of the strategy. Optimization is an ongoing process, involving regular retraining and fine-tuning of models to adapt to changing market conditions.
Real-Time AI for Dynamic Stock Selection
AI models for stock selection can be integrated with real-time data feeds to enable dynamic decision-making. This is particularly useful for traders who require quick reactions to market movements. Real-time AI applications are used to adapt investment strategies instantly based on the latest financial information, market sentiment, and other dynamic factors.
Case Studies: Successful AI-Driven Stock Selection
Detailed Examples of Companies Utilizing AI for Stock Selection
Many firms, from hedge funds to robo-advisors, have successfully integrated AI into their investment processes. These companies use AI to automate the stock selection process, improve their investment returns, and manage risk more effectively. For instance, a leading hedge fund may use machine learning models to predict earnings surprises, while a robo-advisor might leverage NLP to adjust its portfolios based on market news sentiment.
Challenges and Pitfalls
Despite its potential, integrating AI into stock selection is not without challenges. Common pitfalls include overfitting — where the model performs well on historical data but fails to generalize to new data — and model drift — where the model's performance deteriorates as market conditions change. These challenges require careful monitoring, regular model updates, and sometimes even a pivot to new algorithms or data sources.
Future Trends in AI for Stock Selection
Emerging Technologies and Innovations
The field of AI in finance is rapidly evolving. Quantum computing, for instance, promises to unlock new capabilities for processing massive datasets and improving algorithmic predictions. Reinforcement learning continues to advance, potentially leading to AI models that can learn from a broader range of data types. Furthermore, the integration of alternative data sources, such as satellite imagery or social media trends, is becoming increasingly mainstream.
Integration of AI with ESG Factors
Environmental, social, and governance (ESG) factors are gaining importance in investment decisions. AI can help investors integrate these criteria into their stock selection by analyzing company reports, sustainability data, and market sentiment around ESG issues. This not only enhances stock selection strategies but also aligns with increasing investor demand for responsible and ethical investing.
Predictions for the Future of AI in Finance
The future of AI in stock selection appears promising. As AI continues to mature, it will likely become an even more integral part of financial decision-making. Businesses and professionals can expect AI to provide deeper insights into market dynamics, refine investment strategies, and offer more personalized investment advice. The blending of AI with other emerging technologies will only enhance its capabilities, offering new avenues for competitive advantage in the financial markets.
Conclusion
Selecting winning stocks with AI is no longer a futuristic concept but a present reality. For business executives and professionals, leveraging AI can lead to more sophisticated investment strategies, improved risk management, and potentially higher returns. While challenges remain, the benefits of integrating AI into stock selection are clear, making it a valuable tool for modern financial decision-making.
Make AI work at work
Learn how Shieldbase AI can accelerate AI adoption with your own data.