The End of RAG Is Near
Jan 29, 2025
TECHNOLOGY
#rag
A deep dive into why Retrieval-Augmented Generation (RAG) is reaching its limits and what emerging AI retrieval methods—such as RIG, knowledge graphs, and multi-agent systems—mean for enterprise AI strategy.
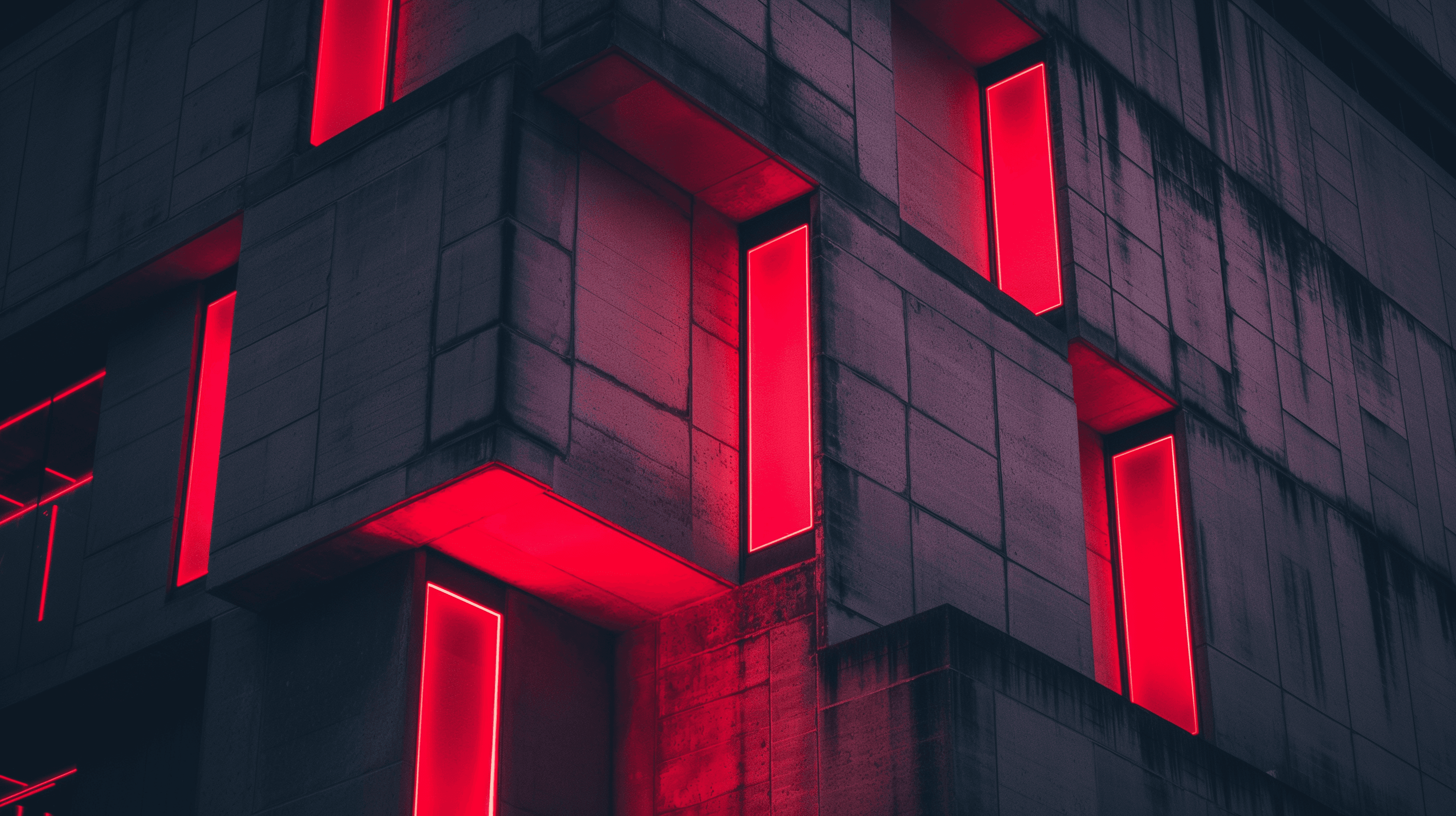
What’s Next for AI Retrieval?
Retrieval-Augmented Generation (RAG) has been a cornerstone of enterprise AI, enabling large language models (LLMs) to fetch external knowledge dynamically and generate more accurate, contextually relevant responses. It was a major step forward in reducing AI hallucinations and ensuring models provided fact-based outputs.
However, as enterprises scale AI adoption, RAG is showing its limitations. Performance bottlenecks, scalability concerns, and cost inefficiencies are leading organizations to question whether it remains the best approach. With newer, more advanced retrieval paradigms emerging, the era of RAG may be nearing its end. So what’s next for enterprise AI retrieval?
The Rise and Limitations of RAG
How RAG Became the Go-To Approach
RAG was developed as a response to one of the biggest challenges in AI—the inability of LLMs to recall information beyond their training data. By integrating external knowledge bases through retrieval mechanisms, enterprises could:
Reduce AI hallucinations by grounding responses in real-world data
Enable real-time updates and dynamic content generation
Enhance enterprise AI applications such as chatbots, research assistants, and legal document analysis
This approach was widely adopted in industries where accuracy and up-to-date information are crucial, such as finance, legal, healthcare, and customer service.
The Cracks in RAG’s Foundation
Despite its advantages, RAG is proving to be a double-edged sword. As organizations deploy AI at scale, they are encountering significant hurdles:
Latency and Performance Issues – Real-time retrieval adds processing overhead, leading to slower response times.
Scalability Challenges – Managing and indexing vast amounts of enterprise data efficiently is complex and costly.
High Infrastructure Costs – Maintaining vector databases and retrieval pipelines requires continuous investment.
Context Window Limitations – Even with retrieval, models are constrained by how much information they can process at once.
Security & Data Governance Risks – Fetching external data introduces compliance risks and potential inaccuracies.
As these challenges compound, enterprises are looking beyond RAG for more efficient and scalable solutions.
What’s Replacing RAG? The Future of AI Retrieval
1. Retrieval-Informed Generation (RIG)
Instead of dynamically fetching external knowledge, Retrieval-Informed Generation (RIG) involves pre-training or fine-tuning models on curated, trusted knowledge bases. This approach:
Reduces inference latency by eliminating real-time retrieval
Improves response consistency by relying on vetted data
Enhances security by keeping sensitive information within controlled environments
Enterprises are experimenting with RIG to build domain-specific AI models that retain up-to-date, high-quality knowledge while improving performance.
2. Knowledge Graph-Powered AI
Structured knowledge graphs offer another alternative, providing well-organized, queryable knowledge that reduces reliance on unstructured data retrieval. With knowledge graphs:
AI models access direct, reliable, and structured facts
Information retrieval becomes more explainable and trustworthy
Compliance-heavy industries can ensure greater data integrity
Enterprises are leveraging knowledge graphs for regulatory compliance, fraud detection, and enterprise search applications.
3. Multi-Agent AI Systems
A more dynamic alternative to RAG is the use of specialized AI agents that collaborate to retrieve, verify, and synthesize information. These multi-agent systems:
Assign different AI models to specific knowledge domains
Validate retrieved data before incorporating it into responses
Use AI orchestrators to manage information flow across systems
This agent-based approach is gaining traction in AI-powered research, cybersecurity, and customer support applications.
4. On-Device and Embedded Knowledge Models
As AI moves closer to the edge, enterprises are exploring ways to embed domain-specific knowledge directly into AI models running on-premises or on devices. Benefits include:
Faster response times by eliminating network-dependent retrieval
Improved privacy and security for sensitive enterprise applications
Reduced reliance on cloud-based knowledge repositories
This approach is particularly relevant for sectors like healthcare, defense, and IoT applications, where real-time AI decision-making is critical.
Implications for Enterprises
With RAG reaching its limits, organizations must rethink their AI knowledge management strategies. Business leaders should consider:
Evaluating Alternative Retrieval Methods – Understanding when to use RIG, knowledge graphs, multi-agent AI, or embedded models.
Investing in Knowledge Curation – Transitioning from raw retrieval to structured and validated knowledge sources.
Optimizing AI Infrastructure – Reducing the overhead of vector databases and retrieval pipelines.
Enhancing Governance and Compliance – Ensuring data accuracy, security, and regulatory alignment in AI-driven decisions.
A hybrid approach may be the best path forward, integrating multiple retrieval and knowledge augmentation strategies tailored to specific enterprise needs.
Conclusion
RAG was an important milestone in AI evolution, but its limitations are becoming more apparent as enterprises scale their AI initiatives. The future lies in more efficient, structured, and dynamic retrieval approaches that balance accuracy, performance, and cost.
As we move beyond RAG, businesses that proactively embrace emerging retrieval technologies will gain a competitive edge in enterprise AI transformation. Now is the time to prepare for the next wave of AI knowledge retrieval.
Make AI work at work
Learn how Shieldbase AI can accelerate AI adoption with your own data.