The Rise of Private AI in Enterprise Deployments
Jan 22, 2025
TECHNOLOGY
#privateai #server
Private AI is emerging as a critical solution for enterprises seeking to balance AI innovation with data privacy and security concerns. This approach involves building and deploying AI solutions within controlled environments, offering benefits like enhanced data security, regulatory compliance, and customized AI models. However, challenges include high initial costs, scalability issues, and the need for specialized expertise.
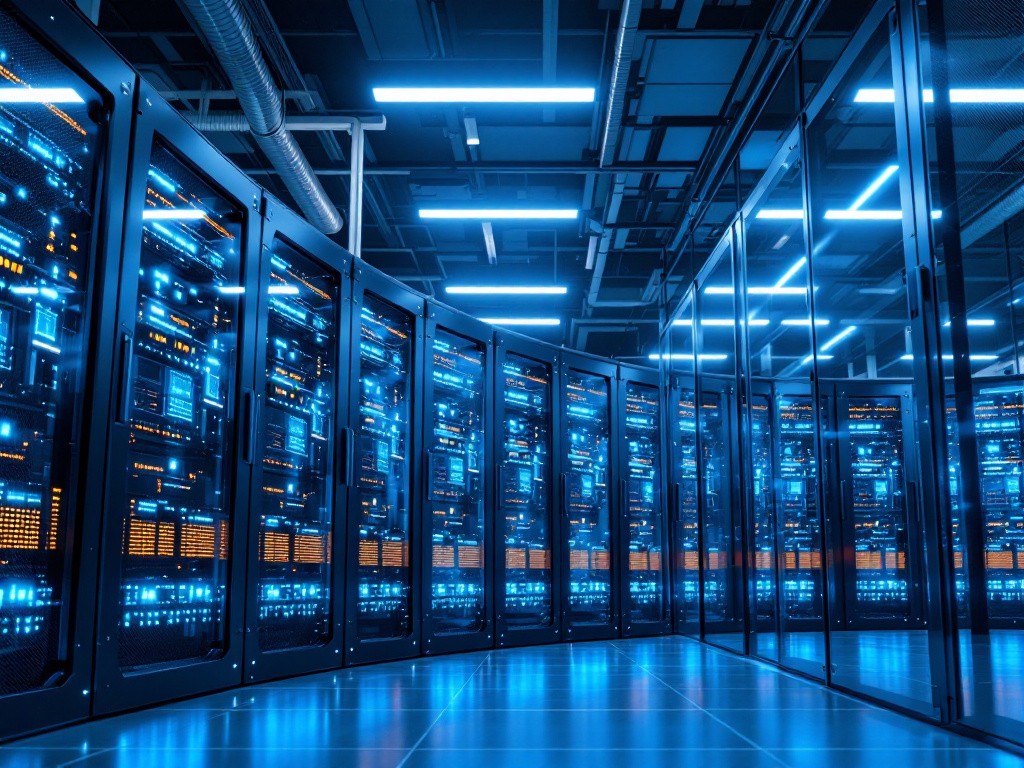
The growing demand for artificial intelligence (AI) is reshaping enterprises, but concerns over data privacy and security are driving a shift toward Private AI. As organizations navigate complex regulatory environments and seek to protect sensitive data, Private AI is emerging as a critical solution for balancing innovation with compliance and security. This article explores the factors fueling the rise of Private AI, its benefits, challenges, and how enterprises can adopt this transformative technology.
Understanding Private AI
Private AI refers to AI solutions that operate within an organization’s controlled environment, ensuring that data remains secure and compliant with regulatory requirements. Unlike public AI models hosted by third-party providers, Private AI is built, deployed, and managed in-house or in hybrid settings, providing unparalleled control over data and infrastructure.
Key characteristics of Private AI include:
Data residency and sovereignty: Ensuring sensitive data stays within defined geographic and legal boundaries.
Enhanced security protocols: Protecting intellectual property and customer data from unauthorized access.
Customization: Tailoring AI models to meet specific industry and organizational needs.
Private AI matters because it addresses fundamental challenges associated with data sensitivity, regulatory compliance, and the need for bespoke AI solutions in industries like healthcare, finance, and government.
Drivers Behind the Rise of Private AI
Several factors are propelling the adoption of Private AI in enterprise deployments:
Data privacy regulations: Laws like GDPR, HIPAA, and CCPA require organizations to maintain strict control over their data, making Private AI an attractive option.
Security concerns: As cyber threats increase, enterprises are prioritizing AI solutions that protect proprietary data and minimize exposure to breaches.
Industry demands: Sectors with unique workflows and compliance needs require AI solutions tailored to their specific challenges.
Advancements in technology: The rise of affordable computing power and edge computing enables enterprises to implement AI solutions on-premises or in hybrid environments.
Benefits of Private AI for Enterprises
The adoption of Private AI offers numerous advantages, including:
Improved compliance: Enterprises can ensure adherence to regulatory frameworks by keeping data within controlled environments.
Data ownership: Organizations retain full control over their data without relying on external platforms.
Customization: AI models can be designed to align closely with business goals and industry-specific requirements.
Reduced latency: On-premises AI deployment accelerates decision-making by reducing reliance on cloud-based processing.
Challenges in Implementing Private AI
Despite its benefits, implementing Private AI comes with challenges:
High initial costs: Building the necessary infrastructure and hiring specialized talent can be expensive.
Scalability: Expanding Private AI solutions while integrating them with legacy systems can be complex.
Technical complexity: Maintaining and updating AI models in-house requires robust technical expertise.
Talent shortage: Finding skilled professionals to develop and manage Private AI systems remains a significant hurdle.
Use Cases of Private AI in Enterprises
Private AI is transforming operations across industries:
Healthcare: Secure analysis of patient data for diagnostics and personalized treatments.
Finance: Fraud detection and risk analysis while ensuring compliance with data protection laws.
Manufacturing: Optimizing production processes using localized AI models.
Retail: Enhancing personalized customer experiences without exposing sensitive consumer data.
How Enterprises Can Get Started with Private AI
Adopting Private AI requires a strategic approach:
Assess organizational needs: Evaluate the feasibility and potential ROI of implementing Private AI.
Build the right infrastructure: Invest in secure servers, edge computing, and robust data pipelines.
Develop or partner for AI models: Decide whether to build in-house solutions or collaborate with trusted vendors.
Ensure security and compliance: Implement robust cybersecurity measures and adhere to data governance standards.
Upskill employees: Train staff to manage and utilize Private AI tools effectively.
Conclusion
Private AI is revolutionizing enterprise deployments by addressing the dual challenge of innovation and security. As industries grapple with increasing regulatory demands and data sensitivity, Private AI provides a path forward for organizations looking to harness the power of AI without compromising compliance or control. Enterprises that proactively align their strategies with this emerging trend will secure a competitive edge in the evolving business landscape.
Make AI work at work
Learn how Shieldbase AI can accelerate AI adoption with your own data.