The Role of AI Accelerators in Enterprise AI Transformation
Oct 27, 2024
ENTERPRISE
#accelerator
AI accelerators are specialized hardware designed to enhance the speed, efficiency, and scalability of AI workloads, enabling enterprises to process complex data and deliver real-time insights critical for competitive advantage.
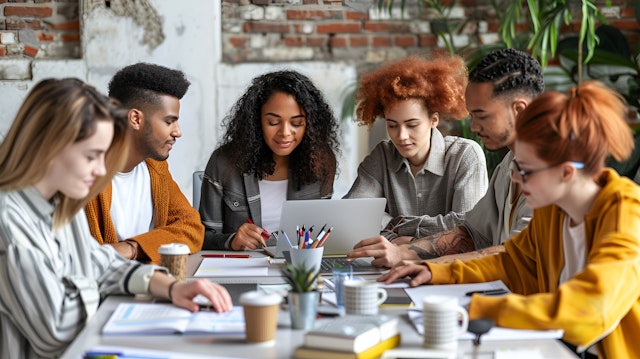
Enterprise AI transformation is reshaping industries by unlocking the power of data and enabling new efficiencies. However, the journey is not without challenges. Modern AI workloads, such as training deep learning models or enabling real-time decision-making, demand immense computational resources. This is where AI accelerators play a pivotal role. These specialized hardware solutions are designed to handle complex AI tasks efficiently, driving the pace and scale of AI adoption in enterprises.
What Are AI Accelerators?
AI accelerators are specialized hardware components built to optimize the performance of AI workloads. Unlike general-purpose CPUs, which are versatile but slower for AI tasks, accelerators are tailored for machine learning and deep learning computations. Examples of AI accelerators include GPUs (Graphics Processing Units), TPUs (Tensor Processing Units), FPGAs (Field-Programmable Gate Arrays), and custom ASICs (Application-Specific Integrated Circuits).
These accelerators excel at parallel processing, making them ideal for handling the vast matrix calculations required for training AI models and performing inference tasks. By leveraging AI accelerators, enterprises can significantly enhance the speed and efficiency of their AI systems.
Why Enterprises Need AI Accelerators
The demand for AI accelerators in enterprises stems from several factors:
Data growth and complexity: Enterprises are generating and consuming more data than ever before. Analyzing this data in a timely manner requires high-performance computing.
Faster insights: Real-time AI applications, such as fraud detection and predictive maintenance, require rapid model inference to deliver actionable insights.
Cost efficiency: While accelerators may have higher upfront costs, they enable faster processing and energy savings, reducing the overall operational expense of AI initiatives.
Industry adoption: Sectors like healthcare, finance, and manufacturing are leading the charge in adopting AI accelerators. For example, GPUs are widely used in genomics for accelerated DNA sequencing, while TPUs power financial risk modeling.
Key Benefits of AI Accelerators in Enterprise AI
AI accelerators provide a range of advantages that make them indispensable for modern enterprises:
Performance gains: Accelerators enable faster training times for large AI models, cutting weeks or months down to days or hours.
Scalability: Enterprises can handle larger datasets and more complex models without compromising on speed or efficiency.
Energy efficiency: AI accelerators are designed to maximize computational throughput while minimizing power consumption, making them environmentally and economically sustainable.
Task specialization: Certain accelerators are optimized for specific tasks, such as natural language processing or computer vision, enhancing accuracy and reliability.
AI Accelerators and Cloud-Based Solutions
The emergence of cloud-based solutions has made AI accelerators more accessible to businesses of all sizes. Cloud providers like AWS, Google Cloud, and Microsoft Azure offer AI accelerator services that allow enterprises to scale their workloads without significant upfront investment in hardware.
Cloud-based AI accelerators: Services like AWS Inferentia and Google Cloud TPUs provide pay-as-you-go access to cutting-edge hardware, making them attractive for experimentation and scaling.
On-premise vs. cloud: While cloud solutions offer flexibility, some enterprises prefer on-premise accelerators for data security, latency, and compliance reasons. Choosing between the two depends on the specific needs and priorities of the business.
Challenges and Considerations
Adopting AI accelerators comes with its own set of challenges:
High upfront costs: Building on-premise infrastructure with AI accelerators requires significant capital investment.
Integration complexity: Integrating accelerators with existing IT systems and workflows can be technically demanding.
Skills gap: Enterprises need skilled professionals who can design, implement, and optimize AI models using these specialized tools.
Vendor lock-in risks: Relying on a single cloud provider’s accelerator technology may limit flexibility and bargaining power.
Emerging Trends in AI Accelerators
The field of AI accelerators is evolving rapidly. Key trends shaping the future include:
Custom silicon development: Companies like Tesla are developing their own AI chips to optimize performance for specific applications.
Edge AI accelerators: Hardware solutions designed for edge devices are enabling real-time AI processing in IoT applications such as autonomous vehicles and smart factories.
Software ecosystems: Open-source frameworks and software stacks are making it easier for developers to leverage AI accelerators effectively.
The Future of AI Accelerators in Enterprise AI
AI accelerators are poised to play an even greater role as enterprises continue their AI transformation. With advancements in hardware and software, these tools will enable new applications in generative AI, multi-agent systems, and beyond. To capitalize on these opportunities, enterprises must:
Assess their current AI infrastructure and identify areas for improvement.
Invest in training and upskilling teams to maximize the value of AI accelerators.
Partner with technology providers to stay ahead of emerging trends and innovations.
Conclusion
AI accelerators are not just a luxury for tech giants—they are becoming a necessity for any enterprise seeking to remain competitive in an AI-driven world. By embracing these technologies, businesses can unlock new levels of performance, efficiency, and scalability in their AI initiatives. The question is no longer whether enterprises should adopt AI accelerators, but how quickly they can integrate them into their transformation strategies.
Make AI work at work
Learn how Shieldbase AI can accelerate AI adoption with your own data.