The Role of Knowledge Graphs in Enterprise AI: Enhancing Data Integration and Semantic Understanding
Mar 22, 2025
TECHNOLOGY
#knowledgegraph
Knowledge graphs enhance enterprise AI by integrating siloed data, improving semantic understanding, and enabling smarter decision-making. By structuring relationships between data points, they power advanced AI applications, enhance search accuracy, and provide greater explainability. As businesses scale AI adoption, leveraging knowledge graphs will be key to unlocking deeper insights and driving innovation.
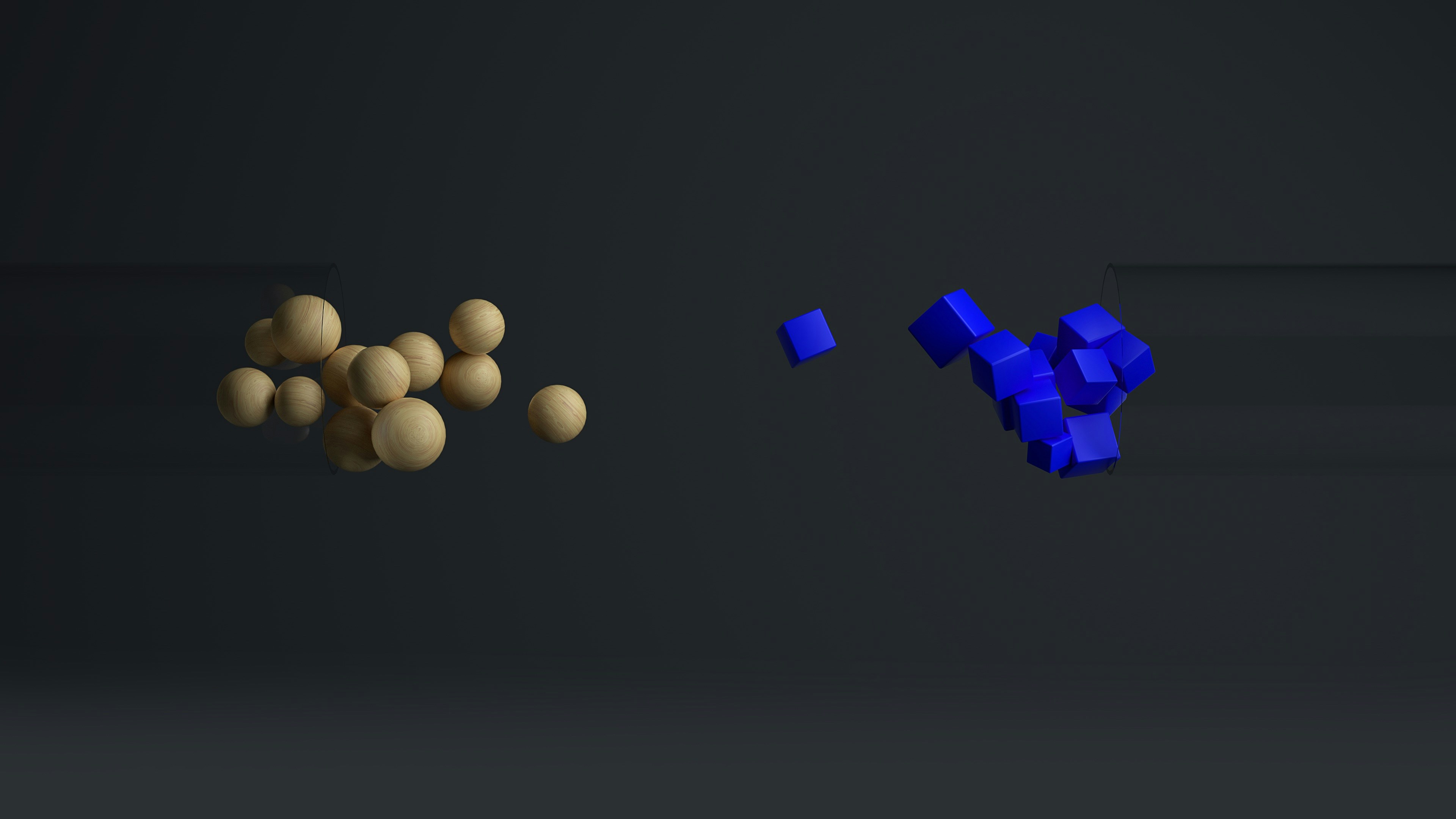
As enterprises continue to embrace AI-driven transformation, one of the biggest challenges they face is integrating vast amounts of structured and unstructured data from multiple sources. Traditional databases and data warehouses struggle to provide the interconnected insights that modern AI applications require.
This is where knowledge graphs (KGs) come in. By structuring information in a way that captures relationships between entities, knowledge graphs enhance data integration, improve semantic understanding, and unlock powerful AI-driven decision-making capabilities.
In this article, we explore the growing role of knowledge graphs in enterprise AI, how they facilitate data-driven innovation, and why they are becoming an essential part of modern AI strategies.
Understanding Knowledge Graphs in Enterprise AI
What is a Knowledge Graph?
A knowledge graph is a structured representation of real-world entities and their relationships. Unlike traditional relational databases, which store data in rows and columns, KGs create a network of interconnected nodes and edges that reflect how data points relate to each other.
For example, in an enterprise setting, a knowledge graph can connect customer profiles, purchase histories, product attributes, and support interactions, allowing AI models to generate insights that go beyond simple data retrieval.
How Knowledge Graphs Differ from Traditional Databases
Relational databases store information in predefined tables, requiring rigid schemas that can be difficult to scale.
Knowledge graphs are dynamic and schema-flexible, allowing enterprises to evolve their data models without major disruptions.
Traditional databases require complex queries to link related data, while KGs use graph-based structures that naturally represent relationships, making queries more intuitive and efficient.
Applications of Knowledge Graphs in Enterprise AI
Enhancing Data Integration Across Silos
Enterprises often struggle with fragmented data spread across CRM systems, ERP platforms, cloud storage, and third-party databases. Knowledge graphs unify these disconnected data sources, providing a holistic view of business information.
For example, a financial services firm can use a KG to integrate customer transaction data, risk profiles, and regulatory compliance information, ensuring AI models have a comprehensive dataset to work with.
Improving Semantic Understanding for AI
Semantic understanding refers to an AI system’s ability to interpret the meaning of data rather than just processing it at a surface level. Knowledge graphs help by encoding relationships between concepts, enabling AI to make more context-aware decisions.
For instance, in a healthcare organization, a knowledge graph can connect symptoms, treatments, drug interactions, and patient histories, allowing AI to provide more accurate diagnostic recommendations.
Powering AI-Driven Search and Recommendations
Many enterprises use knowledge graphs to improve search accuracy and recommendation systems. By understanding relationships between data points, KGs enable AI to provide contextually relevant results rather than just keyword-based matches.
For example, e-commerce platforms leverage knowledge graphs to enhance product recommendations by linking customer behavior, product attributes, and purchase patterns.
Enabling Advanced AI Capabilities
Beyond search and recommendations, KGs play a critical role in advanced AI applications such as:
Conversational AI: Virtual assistants and chatbots use knowledge graphs to understand user intent and provide more natural, context-aware responses.
Fraud Detection: Financial institutions use KGs to detect suspicious patterns by analyzing relationships between transactions, accounts, and known fraud cases.
Enterprise Knowledge Management: Organizations use KGs to structure internal knowledge bases, making it easier for employees to find relevant information and insights.
Benefits of Knowledge Graphs for Enterprises
Faster and More Accurate Decision-Making
By breaking down data silos and providing a structured view of relationships, knowledge graphs enable business leaders to make informed decisions with greater speed and accuracy. AI models powered by KGs can generate real-time insights based on interconnected business data.
Improved AI Explainability
One of the biggest concerns in enterprise AI adoption is the "black box" problem—where AI makes decisions without transparency. Knowledge graphs improve explainability by showing how AI arrives at its conclusions based on the relationships between data points.
Scalability and Flexibility
Enterprises generate massive amounts of data, and KGs provide a scalable solution that adapts as new data sources and relationships emerge. Unlike rigid database schemas, knowledge graphs evolve over time, making them well-suited for dynamic business environments.
Challenges in Implementing Knowledge Graphs
Data Quality and Consistency
A knowledge graph is only as good as the data it integrates. Poor data quality, inconsistencies, and missing relationships can lead to unreliable AI insights. Enterprises must invest in data governance strategies to ensure high-quality, well-structured information.
Complexity in Building and Maintaining KGs
Developing a robust knowledge graph requires expertise in ontology design, data modeling, and graph database technologies. Organizations must carefully plan how they structure relationships to avoid performance issues and ensure scalability.
Integration with Existing AI and Data Systems
Many enterprises already have established AI and data infrastructure. Implementing a knowledge graph requires seamless integration with existing data lakes, machine learning models, and analytics platforms, which can pose technical challenges.
Future Trends in Knowledge Graphs and Enterprise AI
AI-Driven Knowledge Graph Construction
Future advancements will focus on automating knowledge graph creation using AI. Machine learning models will extract entities and relationships from unstructured data sources, reducing the manual effort required to build and maintain KGs.
Industry-Specific Knowledge Graphs
As adoption grows, enterprises will develop industry-specific knowledge graphs tailored to their unique business needs. For example:
Healthcare: Medical knowledge graphs for clinical decision support.
Finance: Risk assessment knowledge graphs for fraud detection.
Manufacturing: Supply chain knowledge graphs for predictive maintenance.
Integration with Multi-Agent AI Systems
Multi-agent AI systems—where multiple AI models work together—will increasingly rely on knowledge graphs for context-aware decision-making. This will enable AI agents to reason, collaborate, and generate insights more effectively.
Conclusion
Knowledge graphs are becoming a cornerstone of enterprise AI, enabling organizations to integrate disparate data sources, improve AI’s semantic understanding, and unlock deeper business insights.
As enterprises continue their AI transformation, investing in knowledge graph technology will provide a competitive advantage by making AI systems more intelligent, explainable, and effective. While challenges exist in implementation, the long-term benefits far outweigh the hurdles.
For business leaders looking to enhance their AI capabilities, now is the time to explore how knowledge graphs can revolutionize data-driven decision-making.
Make AI work at work
Learn how Shieldbase AI can accelerate AI adoption.