Top Challenges Faced by AI Engineers
Nov 14, 2024
TECHNOLOGY
#aiengineers #softwaredevelopment
AI engineers face a range of challenges, from sourcing quality data and managing model complexity to ensuring ethical AI deployment and integrating with legacy systems. Understanding these hurdles is essential for business executives to support their AI teams effectively and drive successful AI transformations within their organizations.
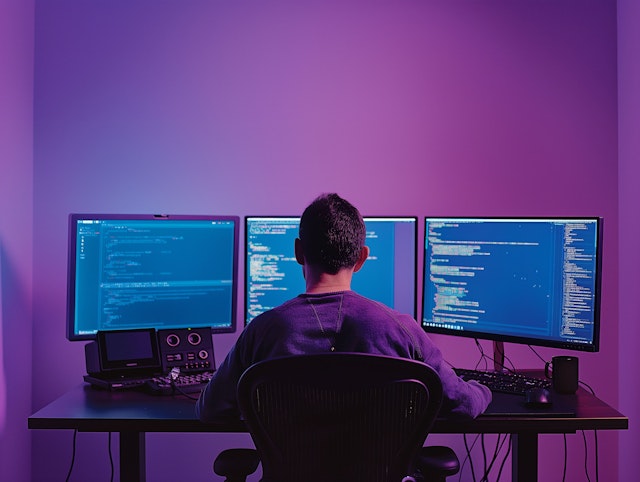
As artificial intelligence (AI) becomes an integral part of business transformation, AI engineers play a critical role in enabling organizations to harness the full potential of AI technologies. However, the journey to successful AI deployment is fraught with challenges. These challenges not only affect the performance and scalability of AI models but also influence how businesses manage their AI initiatives. For business executives and professionals overseeing AI projects, understanding these challenges can help in supporting AI engineers and driving AI initiatives forward.
Data Challenges
AI models are only as good as the data they are trained on. For AI engineers, accessing the right data and managing it effectively are some of the most significant challenges.
Access to Quality Data
The most fundamental challenge AI engineers face is sourcing clean, high-quality data. In enterprise settings, data is often siloed across departments or outdated, leading to difficulties in creating robust training datasets. Without high-quality, labeled data, AI models are prone to inaccuracies and inefficiencies. AI engineers spend a significant portion of their time cleaning, preprocessing, and labeling data before even beginning to train models.
Data Privacy and Compliance
In today's data-driven world, privacy and regulatory compliance are top priorities. AI engineers must navigate a complex landscape of laws like the General Data Protection Regulation (GDPR) and the California Consumer Privacy Act (CCPA), which place strict limitations on how data is collected, stored, and used. Ensuring that AI systems respect privacy while still being able to learn from vast datasets requires careful balancing, often slowing the pace of AI development.
Handling Data Bias
Another challenge that AI engineers must address is data bias. Machine learning models learn patterns from historical data, and if that data is biased, the model will inherently reflect those biases. AI engineers need to be vigilant in detecting and mitigating bias, which can have serious consequences in applications like hiring, loan approvals, and criminal justice. This requires a deep understanding of both the technical aspects of AI and the societal implications of deploying AI systems.
Model Development Challenges
Once data is managed, the next hurdle is building and optimizing the AI models that drive business value.
Model Complexity
AI models, especially in deep learning, can be highly complex. Building a model that strikes the right balance between accuracy and interpretability is a continual challenge. Business executives often demand models that provide high performance, but the complexity of these models can lead to difficulties in understanding how they make decisions. AI engineers must design models that meet the required performance metrics while ensuring they are interpretable enough for stakeholders to trust their results.
Computational Resources
Training large AI models demands significant computational power. For enterprise AI engineers, accessing the right hardware and cloud resources can be a major bottleneck. The cost of high-performance GPUs or cloud computing resources for model training can be prohibitive, especially for smaller businesses. Additionally, engineers must optimize these models to be more resource-efficient, which adds to the complexity of development.
Debugging and Optimization
AI engineers are constantly fine-tuning their models to achieve better performance. Debugging AI models and optimizing them for production can be a time-consuming process. Small errors in model architecture, training parameters, or data preprocessing can lead to suboptimal performance. Engineers must iterate continuously to ensure that their models are not only accurate but also reliable and scalable when deployed in real-world environments.
Deployment and Integration Challenges
Once AI models are built, deploying them into production environments presents its own set of challenges. These issues can impact how well AI initiatives are integrated within an organization’s broader IT infrastructure.
Transitioning from Prototype to Production
AI engineers often face the challenge of transitioning AI models from experimental prototypes to full-scale production systems. Prototypes are typically developed in isolated environments with limited data and resources, which may not accurately reflect real-world conditions. Scaling AI models to handle the demands of enterprise systems requires additional work in terms of stability, scalability, and performance under load. Ensuring that AI models operate effectively in live environments is critical but often challenging.
Integration with Legacy Systems
Many organizations still rely on legacy IT systems, and integrating AI solutions into these systems can be difficult. AI engineers need to ensure that their models are compatible with existing infrastructure, such as databases, APIs, and enterprise resource planning (ERP) systems. This process can be time-consuming and may require significant modifications to both the AI model and the legacy systems it interacts with.
Monitoring and Maintenance
AI models don’t remain static after deployment. Over time, they can experience model drift, where their performance degrades due to changing patterns in data or business environments. AI engineers must establish continuous monitoring frameworks to detect when models are underperforming and implement systems for ongoing retraining. Keeping AI models up-to-date and aligned with business objectives is an ongoing responsibility for engineers.
Collaboration and Organizational Barriers
AI engineering doesn’t occur in isolation. The role of AI engineers often involves significant cross-functional collaboration, but there are organizational challenges that can impede their success.
Cross-Functional Collaboration
AI projects often require collaboration across various departments, including IT, marketing, and business development. However, communication gaps and misaligned goals between business teams and technical teams can delay AI initiatives. AI engineers need to bridge these gaps, ensuring that the AI model they develop aligns with the company’s strategic goals and delivers value to stakeholders.
Lack of Standardized Practices
Another organizational challenge AI engineers face is the absence of standardized best practices for AI development. In many organizations, different teams follow their own processes for building, deploying, and maintaining AI models. This lack of standardization can lead to inefficiencies and inconsistencies, making it harder to scale AI projects across the organization.
Skills Gap
AI is an evolving field, and engineers need to stay up-to-date with the latest advancements in machine learning, deep learning, and other AI technologies. However, many organizations struggle to provide their engineers with the necessary training and resources. The rapid pace of AI development means that AI engineers must engage in continuous learning, but without a strong support structure, they may fall behind.
Ethical and Societal Challenges
The broader societal implications of AI are increasingly becoming a concern for organizations. AI engineers are at the forefront of ensuring that AI systems are built ethically and responsibly.
Building Trustworthy AI
As AI systems are deployed in mission-critical applications, trust in these systems is paramount. AI engineers are tasked with building models that are not only accurate but also transparent and explainable. Business executives must understand that building trustworthy AI is not just about performance but about ensuring that stakeholders—whether customers, regulators, or employees—understand how AI makes decisions and that these decisions are fair.
Balancing Innovation and Ethics
AI technology has vast potential for innovation, but it also raises ethical concerns. AI engineers are often confronted with ethical dilemmas, such as balancing the need for innovation with the potential for misuse. Ensuring that AI systems are deployed responsibly requires careful consideration of both technical and ethical factors, including ensuring that AI systems do not perpetuate harmful biases or infringe on privacy.
Future Outlook
The field of AI engineering is evolving rapidly, and so are the solutions to its challenges. Emerging technologies such as AI accelerators, MLOps (Machine Learning Operations), and federated learning are helping AI engineers address many of these issues. As AI becomes more embedded in enterprise systems, organizations will need to invest in the right tools, resources, and training to support their AI engineers.
Conclusion
AI engineers face a wide array of challenges as they work to develop, deploy, and maintain AI solutions. From managing data and model complexity to ensuring ethical practices, the road to successful AI implementation requires technical expertise, collaboration, and ongoing learning. For business executives, understanding these challenges and supporting AI engineers with the right resources and organizational structures is critical to achieving AI-driven business transformation. By addressing these obstacles, businesses can better navigate the complexities of AI and unlock its full potential.
Make AI work at work
Learn how Shieldbase AI can accelerate AI adoption with your own data.