What It Takes for Data to Become AI Ready
Feb 11, 2025
TECHNOLOGY
#aireadiness #datareadiness
Discover what it takes for data to become AI-ready, from ensuring data quality and integration to leveraging assessment tools and overcoming common challenges, empowering enterprises to drive successful AI initiatives.
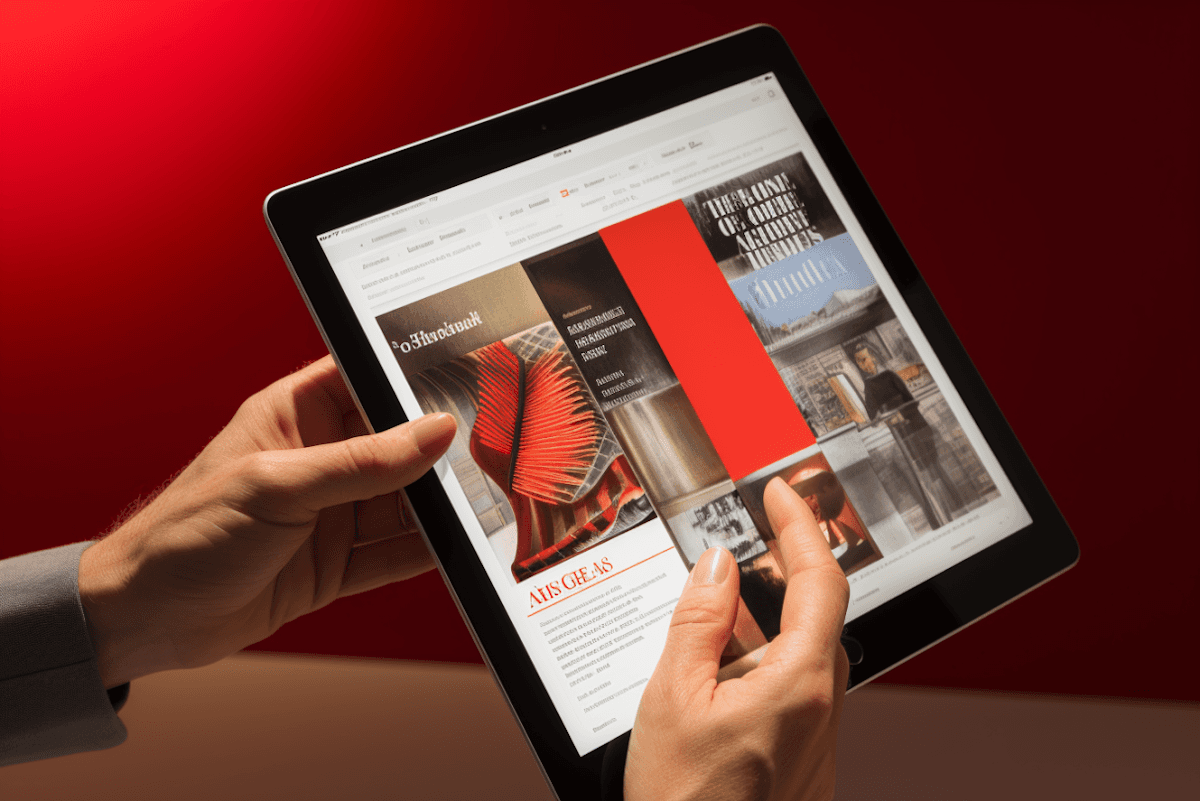
As enterprises race to integrate artificial intelligence (AI) into their operations, the quality of data feeding these AI systems has become a critical success factor. While AI promises transformative insights and efficiencies, it is only as powerful as the data it consumes. For organizations looking to harness AI effectively, ensuring that data is 'AI ready' is a non-negotiable step. This article explores what it takes for data to meet the stringent demands of AI models, offering actionable insights for business executives and professionals.
Understanding AI Readiness
AI readiness refers to the state in which data is prepared, structured, and optimized to be used effectively by AI systems. It involves not only ensuring data quality but also aligning data management practices with the specific needs of AI algorithms. Enterprises often underestimate the importance of this stage, leading to poor AI outcomes, such as inaccurate predictions, biased results, or even project failures. AI readiness encompasses a spectrum of activities, from data cleansing to integration, and is a critical precursor to any AI-driven initiative.
Data Quality and Preprocessing
One of the foundational pillars of AI readiness is data quality. High-quality data is accurate, consistent, complete, and timely. Poor data quality can derail AI projects by introducing noise and inaccuracies into the model's training process.
Techniques for Data Preprocessing
Data Cleaning and Normalization: Removing duplicate, incorrect, or irrelevant data to improve the accuracy of AI models.
Handling Missing Values and Outliers: Applying imputation techniques or excluding problematic data points to enhance data integrity.
Feature Engineering: Transforming raw data into features that better represent the underlying patterns for AI models to learn from.
Data Integration and Management
Modern enterprises deal with data from myriad sources—structured and unstructured, internal and external. Integrating these diverse data streams into a cohesive dataset is critical for AI readiness.
Key Data Management Practices
Data Governance and Compliance: Establishing policies and frameworks to ensure data integrity and compliance with regulations like GDPR and CCPA.
Data Security and Privacy: Implementing robust security measures to protect sensitive data and maintain trust with stakeholders.
Scalability and Accessibility
AI models thrive on large datasets, and as such, data must be scalable to accommodate future growth. Additionally, AI systems need easy access to data across different departments and platforms without facing silos or integration issues.
Best Practices
Use cloud-based storage solutions to handle large volumes of data efficiently.
Implement data cataloging and management tools to improve data discoverability.
Challenges and Future Trends
Preparing data for AI is not without its challenges. Common hurdles include data silos, legacy systems, and lack of data standardization. However, emerging trends such as automated data labeling, synthetic data generation, and AI-driven data management tools are making the journey to AI readiness more manageable.
Conclusion
Data readiness is the unsung hero of successful AI transformations. While AI technologies often capture the spotlight, it is the foundational work of preparing data that determines success or failure. For business executives and professionals, investing in data readiness is not just a technical requirement—it is a strategic imperative that drives competitive advantage in an AI-driven world.
Make AI work at work
Learn how Shieldbase AI can accelerate AI adoption.