What's Next After GenAI
Jun 24, 2024
TECHNOLOGY
#ai #genai #trends
The term "GenAI" has become synonymous with the current state of artificial intelligence (AI) technology. However, as we continue to push the boundaries of AI, it is essential to understand what comes next in the AI landscape. In this article, we will explore the evolution of AI, the rise of explainable AI (XAI), the emergence of human-AI collaboration, the future of AI-driven decision-making, and the impact of AI on the workforce and society.
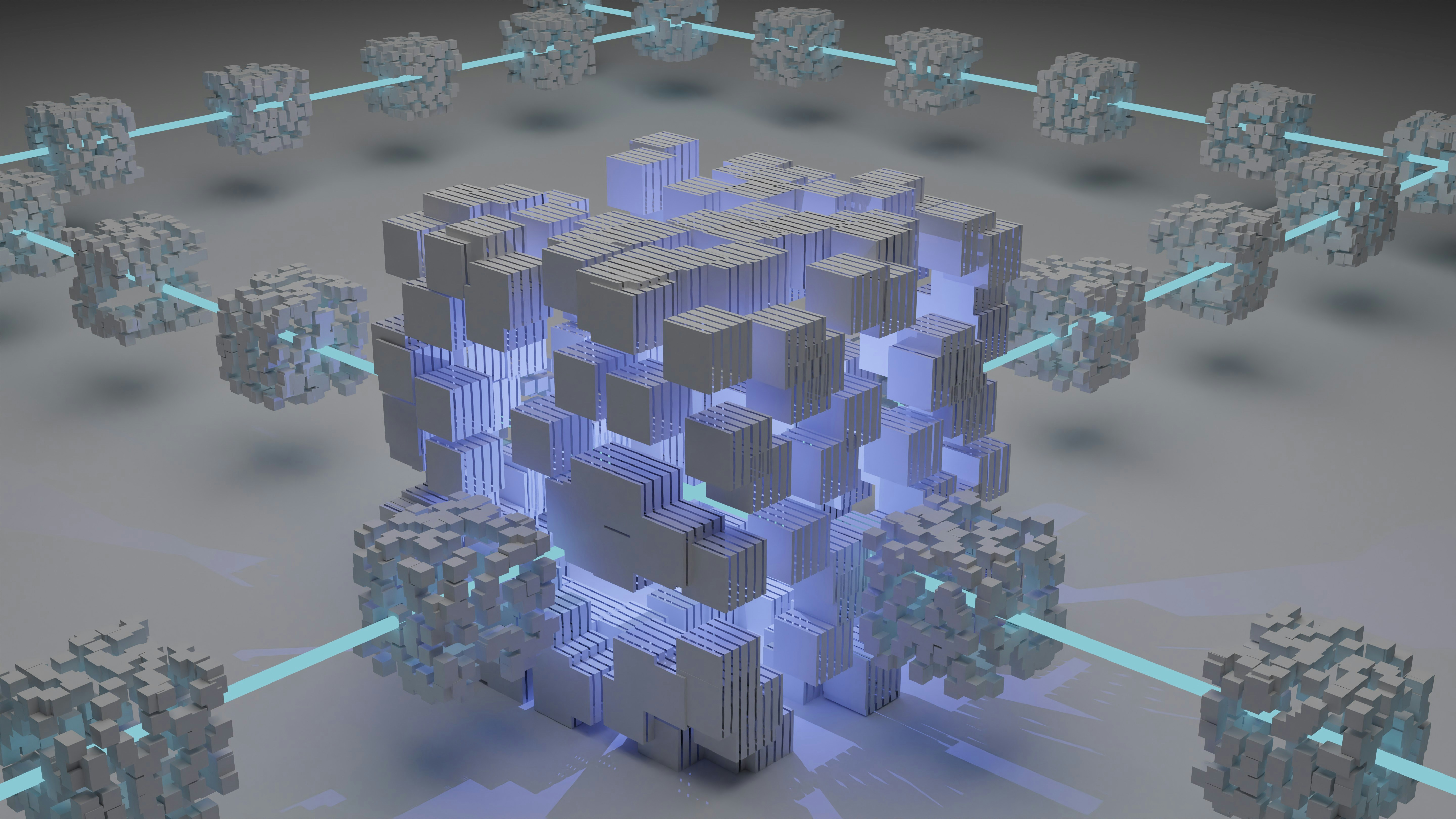
The term "GenAI" has become synonymous with the current state of artificial intelligence (AI) technology. It refers to the general-purpose AI systems that can perform a wide range of tasks, from language translation to image recognition. However, as we continue to push the boundaries of AI, it is essential to understand what comes next in the AI landscape. In this article, we will explore the evolution of AI, the rise of explainable AI (XAI), the emergence of human-AI collaboration, the future of AI-driven decision-making, and the impact of AI on the workforce and society.
The Evolution of AI
The history of AI dates back to the 1950s, when researchers like Alan Turing and John McCarthy began exploring the concept of machines that could think and learn. Since then, AI has evolved through several stages, from rule-based systems to neural networks and deep learning. Each stage has brought significant advancements, such as:
Rule-Based Systems: Early AI systems used predefined rules to make decisions.
Neural Networks: The 1980s saw the development of neural networks, which were inspired by the structure and function of the human brain.
Deep Learning: The 2010s brought the rise of deep learning, which uses neural networks with multiple layers to analyze complex data.
Key Milestones in AI Research and Application
1950s: The first AI program, "Logic Theorist," was developed by Allen Newell and Herbert Simon.
1980s: The development of neural networks by researchers like Geoffrey Hinton and Yann LeCun.
1990s: The introduction of deep learning by researchers like Yoshua Bengio and Hinton.
2010s: The rise of deep learning and its widespread adoption in various industries.
Current Trends and Challenges in AI
Data Quality and Quantity: The need for high-quality and large datasets to train AI models.
Bias and Fairness: Ensuring AI models do not perpetuate biases and are fair in their decision-making.
Explainability and Transparency: The requirement for AI models to be transparent and explainable.
Scalability and Deployment: The challenges of deploying AI models at scale and ensuring they are efficient.
The Rise of Explainable AI (XAI)
Explainable AI (XAI) is a critical development in the evolution of AI. It addresses the transparency and interpretability issues that have long plagued AI systems. XAI provides insights into how AI models make decisions, enabling users to understand and trust the outcomes. The benefits of XAI include:
Improved Trust: Users can understand how AI models arrive at their decisions.
Enhanced Transparency: AI models are transparent, reducing the risk of unintended consequences.
Better Decision-Making: Users can make more informed decisions by understanding the AI model's reasoning.
Applications and Use Cases of XAI
Healthcare: XAI can help doctors understand AI-driven diagnoses and treatment plans.
Finance: XAI can explain AI-driven investment decisions and risk assessments.
Manufacturing: XAI can provide insights into AI-driven quality control and production optimization.
The Emergence of Human-AI Collaboration
Human-AI collaboration is a significant development in the future of AI. It involves integrating AI systems with human expertise to enhance decision-making, creativity, and problem-solving. The benefits of human-AI collaboration include:
Augmented Decision-Making: AI systems can provide data-driven insights, while humans can bring context and intuition.
Enhanced Creativity: AI can generate ideas and concepts, while humans can refine and develop them.
Improved Problem-Solving: AI can analyze complex data, while humans can identify and address the underlying issues.
Examples of Successful Human-AI Collaboration
Healthcare: AI systems can assist doctors in diagnosing diseases, while doctors can provide clinical expertise.
Finance: AI systems can analyze market trends, while financial analysts can make investment decisions.
Manufacturing: AI systems can optimize production processes, while engineers can ensure quality control.
The Future of AI-Driven Decision-Making
AI-driven decision-making is evolving from descriptive analytics (what happened) to predictive analytics (what will happen) and prescriptive analytics (what should happen). This evolution will transform industries like healthcare, finance, and manufacturing. The future of AI-driven decision-making will include:
- **Predictive Analytics**: AI systems will predict future outcomes based on historical data.
- **Prescriptive Analytics**: AI systems will provide recommendations for actions based on predicted outcomes.
- **Real-Time Decision-Making**: AI systems will enable real-time decision-making, enhancing business operations and customer experiences.
The Impact of AI on Workforce and Society
The integration of AI into various industries will significantly impact the workforce and society. The potential benefits and challenges include:
Increased Efficiency: AI can automate repetitive tasks, freeing up human resources for more strategic work.
New Job Opportunities: AI will create new job roles that focus on AI development, maintenance, and integration.
Job Displacement: AI may displace some jobs, requiring retraining and upskilling of the workforce.
Social Benefits: AI can improve healthcare, education, and other social services, enhancing the overall quality of life.
Strategies for Managing the Impact of AI on the Workforce and Society
Upskilling and Reskilling: Providing training programs to help workers adapt to AI-driven roles.
Job Redesign: Redesigning jobs to focus on higher-level tasks that AI cannot perform.
AI Literacy: Educating the workforce and society about AI and its applications.
The future of AI is exciting and full of promise. As we move beyond GenAI, we can expect significant advancements in AI-driven decision-making, human-AI collaboration, and explainable AI. These developments will transform industries and improve the quality of life for individuals. By understanding the evolution of AI and its potential impact, we can better prepare for the future and ensure that AI benefits society as a whole.
Make AI work at work
Learn how Shieldbase AI can accelerate AI adoption with your own data.